The Essential Role of Bioinformatics Data Analysts
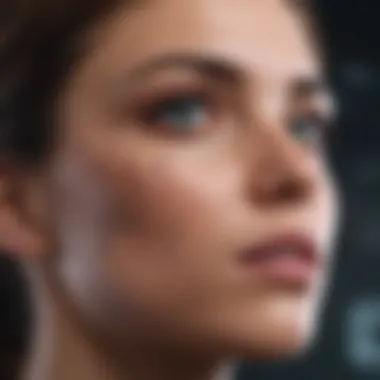
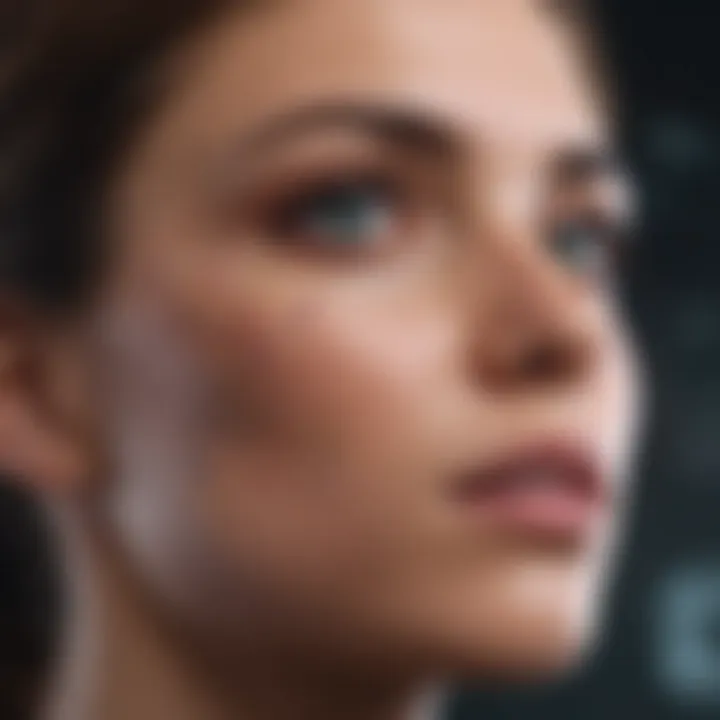
Intro
In the ever-evolving landscape of scientific research, the role of a bioinformatics data analyst has emerged as one of the most crucial components in unraveling the complexities of biological data. With the exponential growth of genomic, proteomic, and other omics data, the need for skilled analysts who can interpret this information has never been more pressing. These professionals stand at the intersection of biology and technology, wielding an arsenal of methodologies and tools that allow them to dissect vast datasets and draw meaningful conclusions.
Understanding their role requires delving into the methodologies they employ as well as the technologies they utilize, both of which play pivotal roles in facilitating sound research and advancing understanding in various fields. The insights they generate not only contribute to basic biological science but also pave the way for practical applications in healthcare, personalized medicine, and beyond.
Methodologies
Description of Research Techniques
At the core of bioinformatics lie diverse research techniques, each designed to handle specific types of data and research questions. Analysts leverage a variety of statistical methods and algorithms to extract patterns that wouldn’t be apparent through traditional means.
- Sequence Alignment: This technique aligns DNA or protein sequences to identify regions of similarity that may indicate functional, structural, or evolutionary relationships.
- Gene Expression Analysis: Using technologies like RNA-Seq or microarrays, analysts determine which genes are active in a given cellular context, providing insights into biological processes.
- Machine Learning: AI-based techniques are becoming increasingly popular in bioinformatics, enabling predictions and classifications that improve as more data becomes available.
Tools and Technologies Used
The toolbox of a bioinformatics data analyst is robust and continuously expanding. Several software solutions and platforms have become industry standards, each offering unique functionalities and capabilities.
- Bioconductor: An open-source software project that provides tools for the analysis and comprehension of high-throughput genomic data.
- Galaxy: A web-based platform that allows users to perform bioinformatics analyses without the need for programming skills.
- R and Python: Programming languages frequently used for statistical analysis and visualization in bioinformatics.
"The integration of computational methods with experimental biology creates a powerful synergy that propels scientific discovery forward."
These tools not only simplify complex analyses but also enhance collaboration across disciplines.
Discussion
Comparison with Previous Research
Historically, bioinformatics was considered a secondary discipline, often overlooked in the shadow of lab-based biology. However, the seismic shifts in data generation techniques have prompted a reevaluation of its importance. Prior research relied heavily on manual data handling, which was both labor-intensive and prone to error. Today, the use of automated tools and algorithms has drastically improved accuracy, allowing scientists to focus on interpretation rather than mere data collection.
Theoretical Implications
The implications of bioinformatics extend beyond mere data analysis. By providing insights into biological processes, it fosters hypotheses generation that can drive future experimental research. This reciprocal relationship between bioinformatics and experimental biology is reshaping our understanding of disease mechanisms, genetic variation, and evolutionary biology.
In exploring the critical role of bioinformatics data analysts, one cannot overlook the far-reaching impacts of their contributions. As the boundaries between biology and technology continue to blur, these analysts are positioned to play an increasingly pivotal role in the ongoing quest for knowledge and innovation in the life sciences.
Understanding Bioinformatics
Bioinformatics stands at the crossroads of biology, computer science, and information technology, making it an essential field for data analysts who work with vast biological datasets. The importance of understanding bioinformatics cannot be overstated; it provides the foundations for analyzing complex data, unlocking biological insights that can lead to significant advancements in medicine, environmental science, and agriculture. Without the principles of bioinformatics, interpreting biological information would often be like trying to find a needle in a haystack, with countless potential insights slipping through the cracks.
This article explores the role of a bioinformatics data analyst and highlights the ways they contribute to the field. Understanding bioinformatics allows these professionals to navigate this intricate web of information competently. The reader will discover how bioinformatics enhances problem-solving capabilities in research and industry alike, thus underscoring its relevance.
Definition and Scope
Bioinformatics can be defined as the science of collecting, storing, analyzing, and sharing biological data using computational tools. It encompasses a broad range of applications, from genomic sequencing to protein structure prediction. The scope of bioinformatics includes:
- Data Analysis: It involves analyzing data from various biological experiments to derive meaningful insights.
- Database Management: Maintaining comprehensive databases to ensure that biological information is accessible for researchers worldwide.
- Algorithm Development: Creating algorithms that can process biological data efficiently.
The field doesn’t simply stop at dense datasets; it pushes the limits of what can be achieved through data-driven insights.
Historical Context
Looking back, the roots of bioinformatics can be traced to the early days of molecular biology and the need for data analysis as sequencing technologies evolved. In the 1970s, the advent of the first sequencing methods prompted scientists to find ways to manage the growing amounts of genetic data. A notable milestone was the launch of the GenBank database in 1982, which laid the groundwork for future projects.
Fast forward to 2001, when the Human Genome Project was completed. This monumental achievement not only represented a monumental effort in sequencing the human DNA but also underscored the importance of bioinformatics in managing and analyzing the vast quantities of data generated.
Interdisciplinary Nature
The interdisciplinary nature of bioinformatics is one of its defining traits. It integrates knowledge from several domains, such as:
- Biology: Understanding biological concepts is crucial for interpreting data accurately.
- Computer Science: Proficiency in programming and algorithm design is required to develop effective analysis tools.
- Statistics: Statistical methods are key in formulating tests and validating observations from data.
Through collaboration, bioinformatics bridges these disciplines, promoting a deeper understanding of biological processes and paving the way for innovations in personalized medicine, genomic research, and environmental sustainability.
"Without the interdisciplinary efforts in bioinformatics, many biological data analyses would face insurmountable challenges."
Understanding bioinformatics is not merely an academic endeavor; it is a practical necessity in a world brimming with complex biological queries, each waiting for a data analyst to decode its secrets.
The Role of a Data Analyst in Bioinformatics
In the realm of bioinformatics, data analysts serve as the backbone of research initiatives. Their work isn't just about crunching numbers. Rather, it is fundamental to making sense of abundant biological information that can lead to groundbreaking discoveries. By interpreting complex datasets, bioinformatics data analysts contribute significantly to fields such as personalized medicine, pharmaceutical development, and environmental monitoring. As biological data continues to proliferate, the significance of data analysts becomes more pronounced, illustrating the growing need for proficient skills in managing and interpreting this data.
Core Responsibilities
The core responsibilities of a bioinformatics data analyst are multifaceted. They begin with data gathering from various sources such as genomic databases, clinical trials, and laboratory experiments. But collecting data is just the tip of the iceberg. Analysts are also tasked with cleaning and organizing data to ensure accuracy and consistency. This step is crucial because messy data can lead to erroneous interpretations, which can stifle potential scientific advancements.
Once the data is prepared, analysts utilize statistical analysis, enabling them to draw insightful conclusions about biological phenomena. They often use tools like R or Python for statistical modeling, ensuring that the results can withstand scrutiny from their peers. The crux of their role, however, is to communicate these findings effectively. This requires not just technical know-how but also a knack for translating complex results into understandable terms for various stakeholders, from biologists to policymakers.
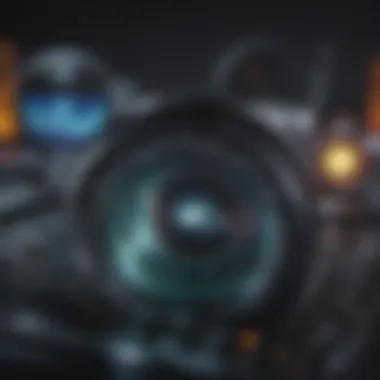
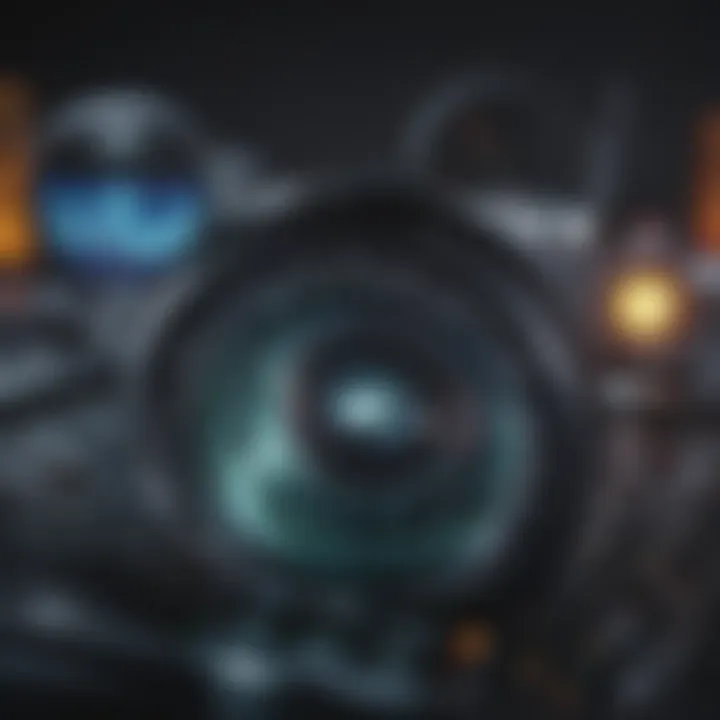
Day-to-Day Tasks
On a daily basis, bioinformatics data analysts juggle a variety of tasks that can vary widely depending on the project at hand. One of the main tasks involves running algorithms that process large datasets, which can be time-consuming but rewarding. For instance, an analyst may work with next-generation sequencing data, looking for mutations that could indicate particular diseases.
Additionally, they frequently collaborate with biologists, geneticists, and other data scientists. This collaboration ensures they are attuned to the biological questions that need answering and can adjust their analytical approaches accordingly. Documentation of data processes and results is another key activity. Proper records not only facilitate repeatability but also allow for peer review, enhancing the overall credibility of the research.
Collaboration with Other Disciplines
Collaborating with other disciplines is another linchpin of the bioinformatics data analyst’s role. They do not work in isolation; their insights must resonate across various scientific realms. For instance, a data analyst might partner with computational biologists to design experiments that address specific hypotheses or work alongside software developers to refine bioinformatics tools.
Moreover, cross-discipline collaboration often includes engagement with medical professionals. Through joint ventures, they can translate data findings into clinical applications, paving the way for advances in healthcare. This multi-faceted approach not only enriches the analyst’s work but also broadens the scope of scientific inquiry.
Bioinformatics data analysts stand as essential players in the team, bridging the divide between vast biological information and practical solutions in medicine and beyond.
Through these core responsibilities, daily tasks, and collaborative efforts, bioinformatics data analysts not only enhance their own professional expertise but also influence the trajectory of research that could have lasting impacts on health and the environment.
Essential Skill Set
The role of a bioinformatics data analyst demands a well-rounded skill set, encompassing a unique blend of analytical abilities, technical know-how, and communication finesse. These proficiencies are not just a feather in the cap; they are the very foundation on which successful analysis rests. Without these skills, interpreting biological data and translating it into actionable insights can feel like trying to find a needle in a haystack.
Analytical Skills
Analytical skills are the bread and butter for anyone in the field of bioinformatics. This includes being able to sift through dense layers of data and spot patterns that might not be obvious at first glance. A data analyst must constantly wear their detective hat, looking for trends and anomalies within huge datasets. Think of it like a treasure hunt where the gems lie hidden among heaps of irrelevant data.
The ability to critically analyze results is paramount. For example, when working on genomic data, one could come across a myriad of variations that could lead to different biological implications. It’s not just about identifying the variations; it’s about understanding their significance. A keen analytical mind can help decipher whether a specific mutation is benign or linked to disease, directing further research effectively.
Technical Proficiencies
In today's tech-driven world, technical proficiencies are non-negotiable for a bioinformatics data analyst. Familiarity with a range of software applications such as Bioconductor, Galaxy, and various R packages is vital. Proficiency in these tools often separates a novice from a seasoned analyst.
Moreover, a solid grasp of programming languages like Python and R is crucial. Being adept in these languages ensures that analysts can fully harness their capabilities to automate tasks and perform complex statistical analyses.
"A bioinformatics analyst is only as skilled as their toolkit allows them to be."
That’s why keeping pace with emerging technologies and languages, such as Julia or Scala for big data processing, often puts analysts a cut above the rest. As the field evolves, those who continue to refine their technical expertise will be the ones leading the charge in innovative research methods.
Communication Skills
Communication skills often find themselves in the background when discussing technical roles. But, in bioinformatics, they are of utmost importance. A data analyst must translate complex results and insights into a language that is digestible by diverse stakeholders, from lab technicians to executive-level scientists. This adaptability is crucial because, while the data holds deep meanings, those meanings must be conveyed clearly and effectively to drive decision-making.
Presenting results through visual aids, reports, or presentations can significantly enhance understanding among team members. The ability to discuss technical challenges with others aids in collaborative problem-solving, fostering an environment where innovative ideas flourish.
In short, a bioinformatics data analyst is not just a data cruncher but a storyteller who brings data to life and illustrates its relevance in the biological context. This blend of analytical prowess, technical skill, and effective communication ensures that the contributions made by these analysts are both profound and impactful, ultimately pushing the boundaries of scientific discovery.
Tools and Technologies
The field of bioinformatics relies heavily on an array of tools and technologies that facilitate effective data analysis and interpretation. In this domain, handling vast and complex datasets is par for the course, and the right tools can make all the difference.
Technologies employed in bioinformatics not only boost productivity but also enhance accuracy and depth of insights drawn from data. Whether it's through sophisticated software applications, robust databases, or versatile programming languages, each element plays a pivotal role in achieving research objectives and advancing scientific discovery.
Software Applications
In bioinformatics, software applications serve as the backbone for various analytical processes. These programs help transform raw data into meaningful information. For instance, applications like BLAST, which finds regions of similarity between biological sequences, allow researchers to predict functionality and evolutionary relationships among genes.
Moreover, tools like Geneious and CLC Genomics Workbench offer user-friendly interfaces that streamline complex tasks such as sequence alignment, phylogenetic analysis, and variant calling. Researchers benefit from integrated workflows that reduce the learning curve and improve efficiency.
- A few critical aspects of software applications include:
- Ease of use: Intuitive interfaces are essential for researchers who may not have extensive programming knowledge.
- Performance: Speed and memory efficiency play vital roles when dealing with large datasets.
- Support and updates: Providers that regularly update their software ensure that users can access the latest functions and bug fixes.
Databases Used in Bioinformatics
Data storage poses its own set of challenges in bioinformatics. Databases act as digital warehouses for a variety of biological data. Major resources such as GenBank and Ensembl provide invaluable insights by housing extensive genomic information.
Utilizing a well-structured database is crucial for effective data retrieval and analysis. Researchers often rely on databases to:
- Store large datasets efficiently
- Retrieve relevant information quickly
- Share data with the larger scientific community
Furthermore, the integration of data from multiple databases allows analysts to perform more comprehensive studies, linking various biological aspects together. For example, integrating The Cancer Genome Atlas (TCGA) with dbSNP can reveal significant correlations between genetic variants and cancer progression.
Programming Languages
Proficiency in programming languages is indispensable for bioinformatics data analysts. Languages like Python, R, and Perl are extensively utilized for scripting and data manipulation. Having a solid footing in programming allows analysts to automate repetitive tasks, thus freeing up time for more analytical work.
- Python is widely favored for its readability and versatility, doubling as both a scripting and a general-purpose language.
- R is particularly strong in statistical analysis and visualizing data.
- Perl, though less common today, still holds its footing in certain niche applications where text processing is crucial.
With the surge of Big Data in biology, a firm grasp of programming also empowers analysts to parse datasets that often defy traditional analytical methods. Ultimately, the fusion of diverse tools and programming languages plays a critical role in the ability of bioinformatics data analysts to uncover new insights and drive innovation.
"The right tools not only simplify the process but also enhance the potential for discovery, enabling researchers to navigate the complexities of biological data efficiently."
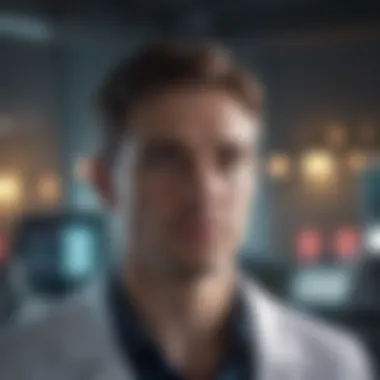
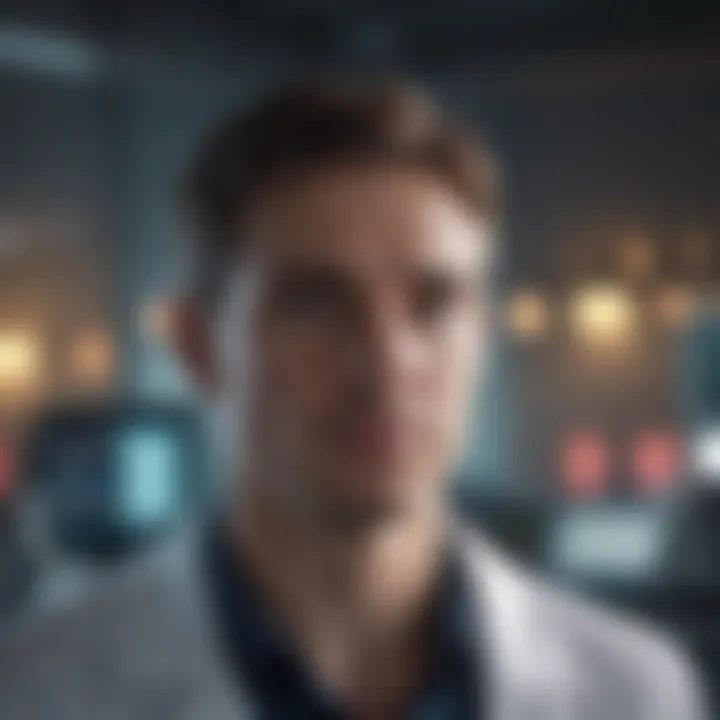
In summary, the collaboration of software applications, databases, and programming languages serves as a foundation upon which bioinformatics research is conducted. These elements together enhance the capabilities of bioinformatics data analysts, ultimately leading to significant advancements within the field.
Data Management Practices
In the field of bioinformatics, effective data management practices are not merely recommendations; they are the backbone supporting every analytical endeavor. The sheer volume and complexity of biological data necessitate a structured approach to handling, processing, and analyzing that data. These practices encompass everything from collecting the initial dataset to preparing, storing, and ultimately retrieving it for analysis. Without these foundational elements, even the most advanced tools and techniques will falter.
Data Collection Techniques
Data collection techniques in bioinformatics start with a clear understanding of the research question at hand. It is not just about gathering numbers; rather, it's about obtaining relevant, high-quality data that can lead to meaningful insights. For instance, researchers often employ methods such as:
- High-throughput sequencing: This involves simultaneous sequencing of many DNA strands, an essential technique in genomic studies.
- Microarray analysis: Used to assess the expression levels of large numbers of genes simultaneously.
- Survey data collection: While not primarily a bioinformatics tool, patient or environmental surveys can provide contextual data that enriches bioinformatic studies.
Each of these approaches requires meticulous planning and execution. Bad data right from the start can lead to flawed analyses, potentially derailing research or resulting in inaccurate findings.
Data Cleaning and Preparation
Once data is collected, the real work begins. Data cleaning and preparation are vital steps that set the stage for effective analysis. This process removes inaccuracies, inconsistencies, and redundant information from datasets. Essential practices include:
- Normalization: Adjusting data to bring it onto a common scale, essential in genomic data analysis.
- Handling missing values: Strategically deciding whether to impute or remove missing entries can significantly impact results.
- Data transformation: Converting data formats or scales to improve the analysis scope and accuracy.
In bioinformatics, overlooking these practices can result in garbage-in-garbage-out scenarios, leading to misinterpretation or incomplete biological truths.
Data Storage and Retrieval
Once data is clean and prepared, efficient storage and retrieval mechanisms come into play. This is where the importance of databases becomes evident. Various approaches exist, from relational databases for structured data to NoSQL databases tailored for unstructured datasets. Key considerations for data storage include:
- Scalability: As research grows, the data management system should be able to expand accordingly.
- Accessibility: Researchers need quick access to their data for analysis and collaboration.
- Security: Protecting sensitive information, especially when dealing with personal health data.
Efficient retrieval methods, including indexed searches and optimized query processes, are crucial for ensuring that valuable time is not wasted during the analysis phases.
Effective data management is the unsung hero in bioinformatics—without it, our insights can easily become obscured by chaos.
In summary, data management practices are critical steps in bioinformatics. Each phase, from collection to cleaning to storage, contributes to the overall health and usability of biological data, ultimately leading to impactful scientific discoveries.
Analytical Approaches
The realm of bioinformatics is a labyrinth of complex data, where the analytical approaches employed are akin to a compass guiding researchers through uncharted territories. Such approaches serve as foundational tools that not only enhance the ability to interpret biological signals but also unravel the intricacies of life at the molecular level. The importance of using proper analytical techniques can't be overstated. The right method can strip away the noise and reveal insights that drive scientific discovery and innovation.
Statistical Methods
Statistical methods play a pivotal role in bioinformatics analysis. When dealing with large datasets, standard practices such as hypothesis testing, confidence intervals, and regression analysis come into play. This is not merely a matter of crunching numbers; it’s about drawing valid conclusions from them.
One significant aspect of statistical methods is their ability to determine correlations. For instance, an analyst might employ correlation coefficients to investigate the relationships between gene expression levels and phenotypic traits. Such analyses can help identify potential biomarkers or therapeutic targets. Modern analytical frameworks like R and Python come equipped with libraries tailored for statistical analysis, making it easier to iterate over data efficiently.
"Statistical rigor allows researchers to substantiate their claims with evidence rather than conjecture."
Another critical statistical method is multivariate analysis, which can handle multiple variables at once. This is essential in bioinformatics, where interactions between genetic variants and environmental factors often determine outcomes like disease susceptibility. Including more complex methods such as ANOVA or PCA can further unveil trends hidden in data.
Machine Learning Techniques
Machine learning is revolutionizing bioinformatics, acting like a lens to magnify patterns in massive biological datasets. With the flexibility to handle different types of data—from structured numerical data to unstructured texts—machine learning techniques can be highly beneficial for predictive modeling in this field.
Common algorithms used include decision trees, random forests, and support vector machines. Each has unique strengths. For example, random forests are excellent for classification problems, such as determining if a patient has a certain genetic disorder based on a host of biomarkers. When applied strategically, these algorithms can manage the variability and complexity of biological data, yielding actionable insights.
Training models can involve cross-validation techniques that ensure the generalizability of findings, a must in healthcare applications. Moreover, neural networks are increasingly used, especially in genomic sequencing and protein structure prediction, marking the dawn of deep learning in the discipline.
Genomic Data Analysis
Genomic data analysis sits as the cornerstone of bioinformatics. The advent of high-throughput sequencing technologies has escalated the volume of data generated, turning the role of the bioinformatics data analyst into that of a data sculptor. Here, the analytical approaches aid in making sense within this monumental data influx.
Fundamental techniques include sequence alignment, variant calling, and annotation. Sequence alignment, for instance, compares the sequences of DNA, RNA, or proteins to identify similarities and differences. Tools like BLAST (Basic Local Alignment Search Tool) are invaluable in this respect, allowing for rapid screening of large sequence databases.
Beyond mere alignment, variant calling algorithms, like GATK (Genome Analysis Toolkit), help identify genetic variations across populations. Knowing how to handle such tools is essential in modern bioinformatics endeavors.
Finally, annotation connects these genomic insights with biological function. It maps genes to their respective functions using databases such as Ensembl or UCSC Genome Browser, bridging the gap between raw data and meaningful interpretation. In summary, embracing analytical approaches is crucial. They form the basis upon which bioinformatics data analysts build, analyze, and extrapolate critical insights in the never-ending quest to understand the complexities of life.
Applications of Bioinformatics Data Analysis
Applications of bioinformatics data analysis are abundant and have far-reaching implications across diverse fields. By leveraging cutting-edge computational techniques and robust data management practices, bioinformatics data analysts help transform raw biological data into meaningful insights that propel advancements in science, healthcare, and environmental studies. Understanding these applications is crucial for students, researchers, and professionals, as they underline the significance of bioinformatics in addressing real-world challenges and enhancing our understanding of biological processes.
Clinical Applications
In clinical settings, bioinformatics plays a pivotal role in personalized medicine. By analyzing genetic information, clinicians can identify specific mutations that might influence treatment plans for individual patients. For instance, consider the use of genomic sequencing in understanding various cancers. A bioinformatics data analyst can sift through heaps of genetic data to pinpoint alterations in genes such as BRCA1 or BRCA2, which are linked to breast and ovarian cancer susceptibility. Similarly, bioinformatics tools are employed to compare patient data against extensive databases, such as those found on Wikipedia, to guide therapy choices that are tailored to a patient’s unique genetic makeup.
Furthermore, predictive models arising from genomic data can lead to earlier diagnosis and improved patient outcomes. Such analyses can help in identifying potential disease outbreaks by scrutinizing gene sequences of pathogens, ultimately leading to better public health responses.
Pharmaceutical Development
In the world of pharmaceuticals, bioinformatics serves as a backbone for drug discovery and development. The process of identifying new drug candidates often involves analyzing enormous datasets related to the interactions between biological molecules. A bioinformatics analyst aids in modeling these interactions, helping researchers understand which compounds bind effectively to target proteins. For example, softwares like Molecular Docking allow analysts to estimate the interaction strength between small molecules and protein receptors.
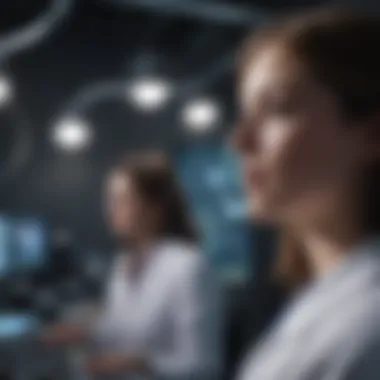
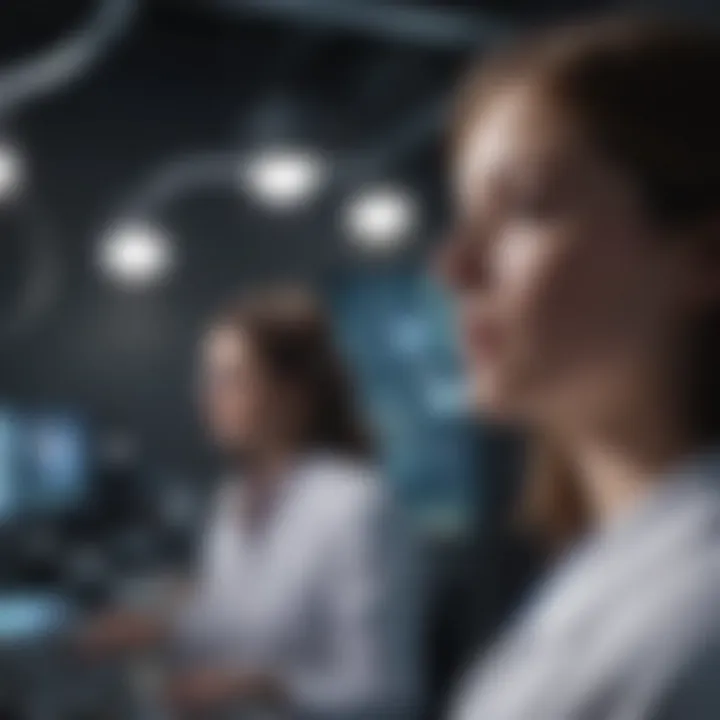
Drug repositioning is another fascinating application that has gained traction recently. This method involves finding new uses for existing drugs, which can save a significant amount of time and resources. Bioinformatics tools can analyze historical data on drug efficacies, allowing analysts to identify potential secondary uses for medications initially designed for different ailments. With the advent of advanced algorithms and high-throughput screening data, the integration of bioinformatics in pharmaceutical development cannot be understated.
Environmental Monitoring
Environmental applications of bioinformatics are becoming increasingly important as society seeks to address ecological challenges. By analyzing genetic data from different species, bioinformatics analysts can help in biodiversity assessments, which are crucial for conservation efforts. For instance, using environmental DNA (eDNA) analysis, researchers can survey aquatic ecosystems without the need for capturing organisms. This approach serves as a non-invasive way to monitor species presence and abundance.
Moreover, bioinformatics helps in understanding the impacts of pollutants on various ecosystems. Analysts can correlate genomic data from affected species with environmental factors to assess how pollutants influence genetic diversity and species resilience. For instance, tracking the genetic responses of fish in polluted waters can provide insight into ecosystem health.
"The integration of bioinformatics into environmental sciences not only enhances ecological monitoring but also supports sustainable practices that could safeguard our planet's biodiversity."
These varied applications highlight how bioinformatics data analysis shapes critical sectors, including healthcare, pharmaceuticals, and environmental science, paving the way for more informed decisions and innovative solutions. In this age of data, bioinformatics data analysts are key players, translating complex datasets into actionable insights.
Challenges in Bioinformatics Data Analysis
In the field of bioinformatics, data analysts play a pivotal role in transforming raw biological data into meaningful insights. Despite the numerous benefits derived from this data analysis, several challenges can pose significant obstacles. These are not just technical issues; they reflect broader concerns that affect the growth and evolution of the field. Understanding these challenges is essential for effectively navigating the complexities of bioinformatics data analysis.
Data Complexity
Data complexity is perhaps the most significant hurdle faced by bioinformatics data analysts. Biological datasets are often massive, intricate, and rife with variability. For instance, a single genomic study can generate terabytes of data, encompassing sequences that vary greatly among individuals. This complexity can obscure patterns, making analysis a formidable undertaking. Analysts must utilize sophisticated methods to filter out noise and extract relevant information from vast datasets.
Moreover, the inherent biological variability introduces another layer of complexity. Individuals of the same species may exhibit vast differences in their genetic codes, influenced by environmental factors and mutations. This variability not only complicates data interpretation but also necessitates the development of tailored analytical approaches. To tackle this, data analysts often employ advanced statistical models or machine learning algorithms, yet the intricacies of biology can still lead to misinterpretations.
"The data in bioinformatics can be like a dense forest; without the right tools and understanding, one can easily get lost."
Integration of Diverse Data Sources
The integration of diverse data sources is another challenge that bioinformatics data analysts frequently encounter. A comprehensive bioinformatics analysis may involve various types of data, including genomic sequences, proteomic data, and clinical records. Each of these data types typically comes from different platforms and formats, making unification a laborious task.
Analysts must often engage with different data management systems that require customized solutions for merging datasets coherently. This can include data harmonization techniques to ensure compatibility. Failure to accurately integrate these data sources can lead to incomplete analyses or misrepresented findings that may misguide further research or clinical applications. Furthermore, the inconsistency of data quality across different sources can greatly hinder analyses, making standardization essential.
Ethical Considerations
Ethical considerations are increasingly critical in the realm of bioinformatics. As data analysts work with sensitive information, especially human genomic data, they must navigate a complex landscape of privacy concerns and ethical dilemmas. Protecting patient anonymity while utilizing and sharing data is paramount; analysts must ensure compliance with regulations such as HIPAA in the United States.
The stakes are particularly high when it comes to informed consent—participants in studies need to fully understand how their data will be used, which can be a daunting task given the technical jargon often involved in bioinformatics. Fostering clear communication between researchers and participants is essential, as any misunderstandings can lead to ethical breaches.
In summary, the challenges faced by bioinformatics data analysts—ranging from data complexity to integration issues and ethical considerations—are multi-faceted and require not just technical proficiency but also a deep understanding of the biological context in which they operate. Addressing these challenges is crucial for unlocking the full potential of bioinformatics, ultimately advancing science and healthcare.
Future Trends in Bioinformatics
The field of bioinformatics is constantly evolving, driven by advancements in technology and the expanding scope of biological research. Understanding future trends in bioinformatics is critical as it shapes not only the methodology of data analysis but also the very nature of scientific discoveries. These trends have implications for personalized medicine, drug discovery, and a host of other applications that touch on human health and environmental sustainability. Given the rapid pace of innovation, staying abreast of these changes is vital for practitioners in the field.
Emerging Technologies
Emerging technologies are redefining what is possible in bioinformatics, providing tools that enhance data management and analysis. One area witnessing significant growth is artificial intelligence (AI). AI techniques, especially machine learning, are increasingly used for pattern recognition in complex biological datasets. For instance, deep learning algorithms allow analysts to predict protein structures from amino acid sequences with remarkable precision.
In addition to AI, cloud computing is transforming data storage and accessibility. No longer constrained by physical hardware, researchers can share and collaborate seamlessly on large datasets. This is particularly important when working with genomic data, which is often massive and evolving at a rapid pace.
Here are a few notable technologies:
- Blockchain for secure data sharing and integrity verification.
- CRISPR gene editing technologies, accompanied by bioinformatics tools for analysis and application.
- Single-Cell RNA Sequencing (scRNA-seq) technologies, enabling detailed insights into cellular heterogeneity.
Personalized Medicine
The concept of personalized medicine is gaining currency, emphasizing that treatment can and should be tailored based on individual genetic profiles. Bioinformatics data analysts play a crucial role in this domain, processing and interpreting genomic data to inform targeted therapies. For example, in oncology, bioinformatics analyzes cancer genomes to identify specific mutations that could be targeted by drugs, thereby optimizing treatment protocols for individual patients.
Moreover, advancements in bioinformatics tools facilitate the integration of genomic data with clinical information, bridging the gap between research and practical medical applications. This trend reflects a broader movement towards preventive medicine, where potential health risks can be identified earlier by examining an individual's genetic disposition.
Big Data Analytics
The phenomenon of big data in bioinformatics should not be underestimated. With the exponential increase in data generation from sequencing technologies and high-throughput experiments, analysts must harness sophisticated data analytics to derive actionable insights. This is where a robust analytical framework becomes crucial, incorporating statistical methods and algorithms for data mining.
The implementation of tools such as Apache Spark and Hadoop allows analysts to process large datasets with improved efficiency. Additionally, effective visualization tools such as Tableau and R can aid in interpreting complex datasets, making it easier for researchers to spot trends and anomalies.
"The best way to predict the future is to create it." - Peter Drucker
As we navigate these exciting trends, it’s crucial that educational institutions adapt their curricula to prepare the next generation of bioinformatics data analysts, ensuring they are well-equipped to meet the challenges ahead.
The End
In reviewing the pivotal role of a bioinformatics data analyst, it becomes clear just how instrumental these professionals are to the ongoing advancement of biological sciences. A conclusion wrapped around the key insights of the article underscores the critical nature of their contributions to data interpretation and management. The blend of analytical and technical expertise equips analysts to sift through massive biological datasets, enabling groundbreaking discoveries that could reshape our understanding of health and disease.
Recapitulation of Key Insights
Here, we'll highlight some of the vital points discussed previously:
- Complex Data Management: Bioinformatics data analysts efficiently handle vast amounts of biological data, ensuring that the integrity and validity of the datasets are maintained. They act as gatekeepers, pinpointing errors and anomalies that could skew research outcomes.
- Integration of Diverse Disciplines: The interdisciplinary nature of bioinformatics requires analysts to work closely with experts in genetics, molecular biology, and computational science, fostering a collaborative environment that enhances overall research quality.
- Emerging Technologies and Tools: A keen understanding of the latest tools, including programming languages like Python and software such as R, arms analysts with the capability to perform advanced data analyses that push the boundaries of scientific knowledge.
- Ethical Considerations: With advancements come responsibilities. It’s paramount that bioinformatics analysts also navigate the ethical landscapes concerning data privacy and the implications of their findings on healthcare policies.
The Importance of Ongoing Research
The field of bioinformatics is ever-evolving, and continual research is crucial to keep pace with technological advancements and emerging biological discoveries. Here are a few reasons why ongoing research remains vital:
- Innovation in Methodologies: As new data types emerge, methodologies must be developed or refined. Continuous research facilitates the discovery of novel analytical techniques that can yield deeper insights into complex biological systems.
- Adaptation to Changing Technologies: Technology shifts rapidly; ongoing research allows bioinformatics data analysts to adapt their skills and toolsets to new platforms and software, keeping their analyses relevant and robust.
- Bridging Knowledge Gaps: Research paves the way for new hypotheses and theories. By examining existing datasets and addressing unanswered questions, bioinformatics analysts contribute to a more complete scientific narrative.
- Enhancing Clinical Applications: Ongoing research in bioinformatics leads to improved diagnostic tools and personalized medicine approaches, directly impacting patient care and treatment efficacy.
- Fostering Interdisciplinary Relationships: Collaboration with other research areas encourages cross-pollination of ideas, leading to richer, more holistic approaches to scientific challenges.
In summary, the role of a bioinformatics data analyst is multifaceted, embodying a critical intersection of biology, technology, and ethics. As we continue to uncover the intricacies of biological data, the need for skilled analysts will grow, making their work vital in shaping the future of science.