AlphaFold Online: Transforming Protein Structure Prediction
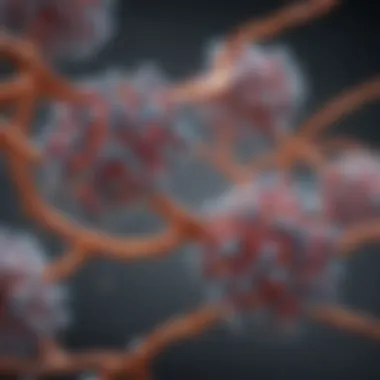
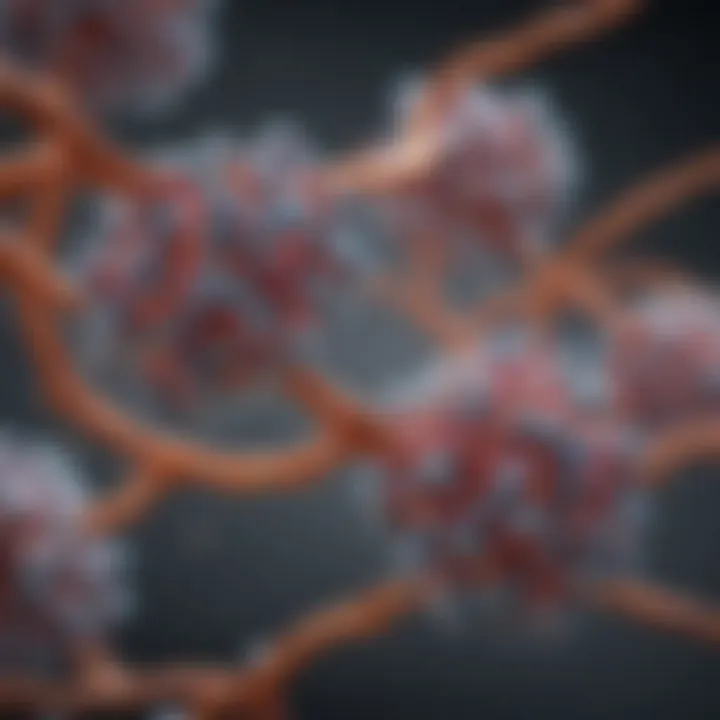
Intro
The exploration of protein structures has long been a cornerstone of molecular biology, bearing implications that stretch across medicine, genetics, and biotechnology. Here enters AlphaFold Online, a revolutionary tool transforming our approach to understanding protein folding. Developed by DeepMind, AlphaFold leverages advanced algorithms and machine learning techniques to predict protein structures with unprecedented accuracy. This advancement not only enhances our comprehension of biological processes but also holds promise for significant breakthroughs in drug discovery and disease understanding.
As researchers, educators, and students dive into the intricacies of AlphaFold, it becomes crucial to understand not only how the system works but also the practical applications that stem from its results. This article aims to dissect the methodologies employed by AlphaFold, delve into relevant discussions comparing its findings to previous research, and examine theoretical implications while offering insights into ethical considerations.
The unfolding narrative provides a thorough understanding of both the mechanics and the utility of AlphaFold Online, making it an essential read for those engaged in the life sciences.
Methodologies
Description of Research Techniques
At the heart of AlphaFold's functioning lies a meticulous blending of various research techniques. It utilizes neural networks to decode the complex, often ambiguous nature of protein folding. A core aspect involves training on vast datasets, which include known protein structures and sequences. This enables the algorithm to grasp patterns that govern how amino acids interactions dictate the final three-dimensional shape of the proteins.
In particular, AlphaFold employs:
- Recurrent Neural Networks (RNNs) to capture sequential data from protein structures.
- Attention Mechanisms that help the model focus on relevant parts of the protein sequence, mimicking the way humans focus on key details.
- Graph-based Learning to represent protein structures as graphs of nodes and edges, which reveal how different parts of the protein communicate with each other.
Tools and Technologies Used
To facilitate its complex predictions, AlphaFold integrates several cutting-edge technologies. Notably, its engine runs on sophisticated hardware, often harnessing the power of Graphics Processing Units (GPUs) or Tensor Processing Units (TPUs) to increase processing speeds substantially. The combination of robust computational power and innovative algorithms allows AlphaFold to deliver profound results efficiently, making previously time-consuming tasks feasible at an unprecedented pace.
"AlphaFold doesn't just predict protein structures—it redefines the narrative of what we thought was possible in bioinformatics."
Discussion
Comparison with Previous Research
When set against the backdrop of traditional methods in protein structure prediction, AlphaFold's capabilities shine even brighter. Prior to AlphaFold's advent, methodologies relied heavily on physical methods like X-ray crystallography, which, while often accurate, demanded significant time and resources. Algorithms such as homology modeling provided a more rapid approach, but their predictions were less reliable when faced with sequences lacking similar templates.
This gap in capabilities is precisely where AlphaFold excels. Its accuracy, often rivalling experimental techniques, has led to a paradigm shift in how researchers approach structural biology. Now, rather than being a supplementary tool for experimental validation, AlphaFold can act as a primary research resource, altering project designs and experimental frameworks in critical ways.
Theoretical Implications
The repercussions of AlphaFold's performance extend into theoretical realms as well. Understanding protein folding not only throws light on fundamental biological processes but also opens up avenues for comprehension of molecular pathologies like Alzheimer's and Parkinson's diseases. The more accessible and accurate predictions provided by AlphaFold stand to redefine hypotheses regarding protein behavior, particularly in identifying potentially malfunctioning structures before they lead to diseases.
As one looks to the horizon of scientific discovery, the prospects are indeed vast. AlphaFold has the potential to forge connections between disparate fields of study, aiding in cross-disciplinary research and fostering collaboration that ultimately drives innovation and understanding.
In summary, as AlphaFold Online becomes entrenched in the scaffolding of modern research, an integral aspect will be recognizing its role and contributions. Understanding its methodologies, comparing its accuracy to historical approaches, and appreciating its theoretical implications could lead to transformative changes across biology and medicine.
Preface to AlphaFold
AlphaFold stands at the forefront of modern bioinformatics, and its significance cannot be overstated. Delving into this subject is crucial, especially as researchers and scientists now stand on the brink of unprecedented advancements in understanding protein structures. This introduction will cover the importance of grasping these foundational concepts and set the stage for an in-depth exploration of AlphaFold’s intricate capabilities and applications.
Overview of Protein Folding
Protein folding is not just a biological curiosity; it is the very core of how living organisms function. Proteins, composed of long chains of amino acids, must fold into specific three-dimensional shapes to perform their roles efficiently. Think of it like origami: a flat piece of paper can be transformed into intricate shapes, but if the folds are incorrect, the result can be a mess. Similarly, misfolded proteins can lead to severe diseases, such as Alzheimer's and Parkinson's.
Understanding protein folding is pivotal for numerous scientific fields, including pharmacology and biotechnology. The chance to predict and visualize these structures regularly transforms our approach to medicine and therapeutics. It’s like having a key that opens many doors to potential cures and analyses. The way proteins unfold, misfold, and refold can reveal much about cell processes and signal pathways.
Significance of AlphaFold in Modern Science
AlphaFold’s rise marks a watershed moment in scientific research. The tool developed by DeepMind employs cutting-edge machine learning strategies, enabling it to predict protein structures with remarkable accuracy. The implications of this are far-reaching:
- Accelerated Research: AlphaFold can vastly reduce the time required for structure determination, giving researchers more bandwidth to focus on experimental validation or other aspects of their work.
- Enhanced Drug Development: By providing accurate structural models, AlphaFold helps in identifying potential drug targets swiftly and developing effective therapeutics against a myriad of diseases.
- Boosting Collaboration: As AlphaFold is accessible online, it fosters collaboration across disciplines and institutions—think of it as a communal library where anyone can access crucial information.
In essence, AlphaFold acts as a catalyst, propelling us toward innovations that can reshape our understanding of biology, enhance medical methodologies, and ultimately ensure a healthier future. Its introduction is not merely an incremental step but rather a giant leap towards mastering the complexities of molecular science.
Foundational Principles of AlphaFold
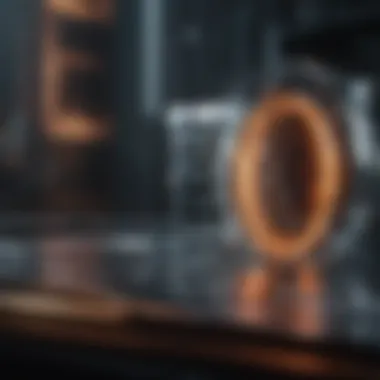
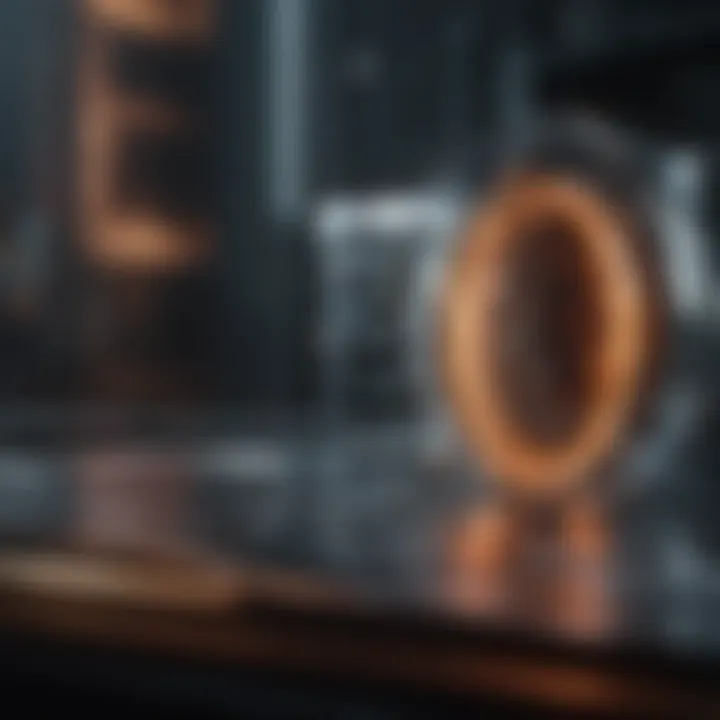
The significance of the foundational principles of AlphaFold cannot be overstated. This section delves into the core concepts that drive AlphaFold’s remarkable ability to predict protein structures. Understanding these principles equips users—from students to seasoned researchers—with the knowledge needed to effectively utilize this innovative tool, highlighting both its potential and limitations.
Machine Learning and AI in Protein Prediction
At the heart of AlphaFold's functionality is a sophisticated application of machine learning and AI. These technologies allow the model to learn from an extensive dataset of known protein structures, identifying patterns that govern how proteins fold. Instead of relying solely on traditional biophysics, AlphaFold turns to deep learning techniques which enable it to predict a protein's 3D structure from its amino acid sequence with astonishing accuracy.
This approach not only accelerates the prediction process but also improves the reliability of results compared to earlier models. AlphaFold uses a neural network that has been trained on a variety of sequences and structures. The ability to draw on this vast well of data means that it can take into consideration the nuances of different proteins, which might go unnoticed in more simplistic prediction methods. As a matter of fact, the model's predictions have been shown to surpass those made by many other protein structure prediction tools, placing it on the cutting edge of bioinformatics.
Neural Networks in AlphaFold
The architecture of AlphaFold leverages deep neural networks, a type of AI modeled after the human brain. Typically, this involves multiple layers of interconnected nodes that process information in a manner akin to neuronal activity. Each layer of the network can learn increasingly abstract representations of the data, enabling AlphaFold to make sense of intricate relationships within the protein sequences.
In practical terms, the neural networks dissect the sequential data of amino acids and assess how they correlate with possible spatial arrangements. AlphaFold's architecture adopts a technique known as the attention mechanism, which allows the model to weigh the importance of different parts of the input sequence when making predictions. This not only improves accuracy but also leads to insightful predictions regarding protein interactions and functions, broadening its applicability across various scientific disciplines.
Understanding the Data Input Requirements
To harness the power of AlphaFold, users must be cognizant of the data input requirements—what the system necessitates in order to produce reliable results. Typically, AlphaFold requires a protein sequence represented in a standard format, such as FASTA, which outlines the linear arrangement of amino acids. However, it's not just a matter of throwing any random sequence into the tool; quality matters.
- Sequence Quality: Ensure the sequences are clean and error-free. An unrefined sequence can lead to flawed predictions, much like trying to build a house on a shaky foundation.
- Related Sequences: Including homologous sequences helps AlphaFold draw from evolutionary data, making predictions more robust. Learning from related proteins can significantly enhance the model's understanding of potential conformations.
- Contextual Information: Providing additional biological context or experimental data when available can fine-tune the output, leading to insights that are more aligned with empirical observations.
In summary, proficiently utilizing AlphaFold mandates an awareness of how to prepare and input data correctly, laying the groundwork for effective structure prediction. Understanding these foundational principles equips users to not only leverage AlphaFold's capabilities but also appreciate the underlying mechanics that power its predictive prowess.
Using AlphaFold Online
AlphaFold online stands at the forefront of computational biology, unlocking vast potentials in the realm of protein structure prediction. The accessibility and user-friendliness of this platform make it a vital resource, particularly for students, researchers, educators, and professionals aiming to dive into the intricate world of proteins. Understanding how to effectively use AlphaFold online can lead to significant advancements in various scientific fields, from drug discovery to structural biology.
Accessing the Platform
Getting started with AlphaFold online is relatively straightforward. The first step involves visiting the official DeepMind AlphaFold webpage, where the portal is housed. Once the web page is loaded, it’s crucial to create an account by providing some basic information. This often includes an email address and establishing a password.
- Why is this important? Creating an account not only secures your work but also helps in tracking the analysis you conduct over time. Furthermore, this account can offer access to updates and resources that are helpful in navigating the platform.
After account creation, users should familiarize themselves with the platform's requirements. Internet speed, for instance, may alter upload times and computational efficiency during prediction tasks. Having a robust and stable connection can enhance the overall experience, especially when dealing with complex sequences.
Navigating the User Interface
Once logged in, navigating AlphaFold's user interface presents a blend of simplicity and sophistication. The layout is designed for ease of use, but it holds numerous tools that can appear overwhelming at first glance.
- At the top of the page, you'll find a navigation bar which includes options like
- The central part of the interface is dedicated to input fields where users can enter protein sequences in FASTA format or upload files directly.
- Home
- Help
- Contact
These sections are beneficial for brushing up on functionalities or seeking support if any issues arise.
Visual aids are provided throughout, guiding users in the correct format for input. A pop-up tutorial might also offer tips as one explores various functionalities, ensuring no one wanders aimlessly through the process.
Uploading and Managing Sequences
The aspect of uploading sequences is where AlphaFold's utility truly shines. After navigating to the sequence upload section, users can either copy and paste their sequences or upload a file. Once this step is completed, one might need to specify additional parameters that could refine the predictions.
- Managing sequences:
- Organize your sequences into groups, which can help in batch analysis.
- Regularly review and update your data as necessary; keeping a clean workspace enables a smoother workflow.
After the upload, alpha fold churns through the sequence, using advanced algorithms to predict the protein structure. Results are displayed clearly on a dedicated results page, which is easy to interpret.
To summarize, efficient use of AlphaFold online starts from a simple yet secure account creation to navigating its tools proficiently. As researchers and professionals delve deeper into protein science, the ability to manage sequences effectively becomes essential. Not only does this ensure accurate predictions, but it also allows users to leverage the full capabilities of this powerful technology.
"Navigating through a new tool can feel like trying to find your way in a dense forest without a map. But with practice and exploration, one will carve pathways leading to substantial discoveries."
Understanding AlphaFold's Predictions
The growing significance of AlphaFold in biomedicine and structural biology hinges on its ability to predict protein structures accurately. Understanding these predictions can transform the scientific landscape, enabling breakthroughs that were previously thought unattainable. As we dissect AlphaFold's performance, we encounter various dimensions that demand attention: precision in results, the user experience when interpreting those results, and the relevance of visualizing protein structures.
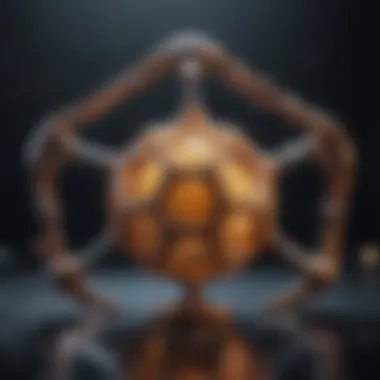
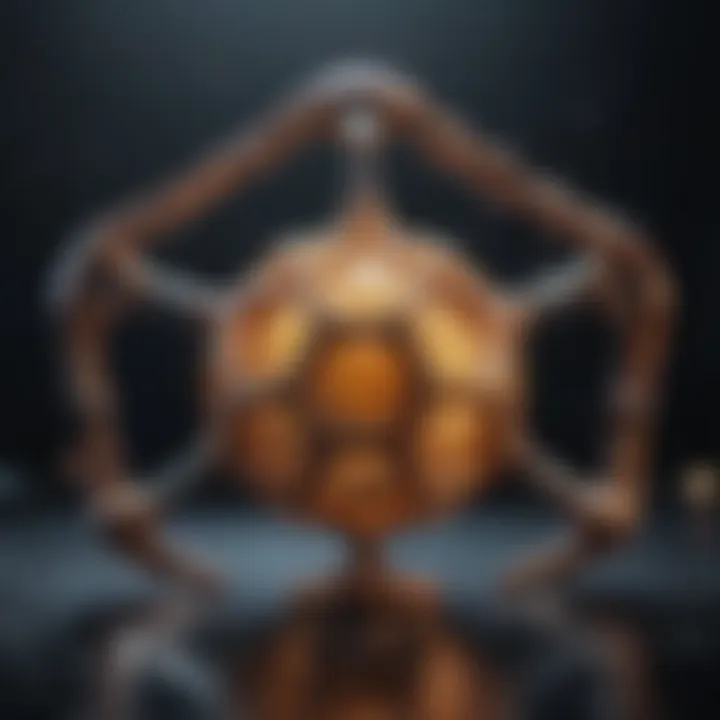
Interpreting Result Outputs
Interpreting the outputs generated by AlphaFold is not just a matter of reading numbers or charts; it is about understanding biological implications that stem from these results. When AlphaFold produces a predicted protein structure, it provides several outputs, such as confidence scores, which indicate the reliability of the predicted structure.
This confidence is often encapsulated in a metric known as the pLDDT score, which stands for predicted Local Distance Difference Test. The range of this score runs from 0 to 100, with higher numbers suggesting more reliable predictions. For instance, a protein structure with a pLDDT of 90 or above signifies that researchers can trust the model’s predictions with decent confidence.
In practical terms, these scores help researchers differentiate between critical regions where detailed study is warranted and others that may be less significant. Correctly interpreting these outputs can lead researchers to formulate hypotheses, design further experiments, or refine existing strategies accordingly. One significant advantage here is that it empowers experimental biologists to prioritize their resources effectively, focusing on structures that promise deeper insights into biological processes or disease mechanisms.
Visualization of Protein Structures
Visualizing protein structures predicted by AlphaFold adds another layer of understanding that raw data cannot provide. A good picture is worth a thousand words, and in this case, it’s worth understanding complex molecular interactions. AlphaFold provides the 3D coordinates of atoms within proteins, creating digital models that can be visualized using software tools—like PyMOL or Chimera.
The visualization aids researchers in seeing how changes in structure may affect function. It opens the door to understanding protein dynamics: how proteins fold, interact with other molecules, and respond to environmental changes—all of these are crucial factors in areas such as drug development and genetic engineering.
There are several methods through which protein structures can be visualized effectively. Here’s a short overview of common approaches:
- Ribbon Diagrams: These depict the protein's backbone and secondary structures (like alpha-helices and beta-sheets) in a simplified way.
- Surface Representation: This visualization helps understand protein interactions by displaying the molecular surface, highlighting potential interaction sites.
- Atomic Representation: This provides a more detailed look at specific amino acids and can aid in pinpointing mutations or active sites critical for interactions with ligands or enzymes.
"Visualization is the bridge between dry data and scientific discovery, making the invisible, visible."
Applications of AlphaFold in Research
The advent of AlphaFold marks a transformative phase for protein structure prediction, with implications stretching far beyond just theoretical biology. It is crucial not just for the scientific community but also for the pharmaceutical industry, genetic research, and many other fields. This section discusses key applications where AlphaFold demonstrates its prowess and utility, highlighting specific elements and benefits.
Role in Drug Discovery
In drug discovery, identifying the correct protein target is a critical step. The ability of AlphaFold to predict the 3D structures of proteins with exceptional accuracy aids in this process significantly. For instance, when tackling complex diseases like cancer, knowing the precise structure of a target protein allows researchers to design molecules that fit into binding sites more effectively.
One practical application can be seen in the development of inhibitors against specific enzymes. By accurately mapping out the structure, researchers can employ virtual screening techniques to identify potential lead compounds. It’s like having the best map before embarking on a treasure hunt. Without AlphaFold, researchers often had to rely on homology modeling or X-ray crystallography, both of which have limitations, such as time consumption and accuracy issues.
Contributions to Structural Biology
AlphaFold's contributions to structural biology are nothing to sneeze at. Structural biologists have faced challenges in elucidating the structures of many proteins, especially those that are membrane-bound or difficult to crystallize. AlphaFold offers a bridge where experimental methods fall short. For example, consider the case of G-protein-coupled receptors (GPCRs). These proteins are notoriously difficult to study but play essential roles in pharmacology. By providing accurate predictive models, AlphaFold helps researchers comprehend how these proteins function at the molecular level, which can lead to breakthroughs in targeted therapies.
Moreover, AlphaFold doesn't just predict structures; it also resonates with evolutionary insights. By analyzing predictions across different species, scientists can uncover conserved mechanisms, helping to illuminate pathways that might be elusive through traditional structural analysis. This capability greatly enhances both the educational and research landscapes.
Insights into Genetic Disorders
Genetic disorders are often linked to protein misfolding or mutations affecting protein structure. AlphaFold plays a pivotal role in understanding these complex relationships. For instance, by predicting the structure of altered proteins, researchers can identify why certain mutations lead to disease states. An example would be cystic fibrosis. In this case, the mutation in the CFTR gene leads to improper folding of the protein, causing the disease. Understanding the structural implications of this mutation through AlphaFold could lead to targeted therapeutic strategies.
Furthermore, studying rare genetic disorders can be remarkably enhanced. For instance, many of these disorders involve unique mutations that disrupt protein folding and function. AlphaFold's predictive ability allows researchers to simulate various scenarios involving these mutations, potentially leading to innovative treatment approaches and patient-specific therapies.
The insights from AlphaFold can dramatically shift the paradigm in how genetic disorders are conceptualized and treated, enabling precision medicine to evolve.
Overall, AlphaFold's applications extend deeply into various areas, demonstrating its significance and the promising future it holds. As researchers continue to explore its full potential, the world stands to gain greatly from more tailored and effective treatments stemming from understanding protein structures.
Comparative Analysis with Other Prediction Tools
In the landscape of bioinformatics, the emergence of tools for protein structure prediction has drastically transformed research methodologies. Increased interest in computational biology has led to the development of numerous platforms, making it essential for researchers to conduct thorough comparative analyses among these tools. In this section, we examine AlphaFold and its competitors to showcase the specific elements and considerations that underline the importance of such comparisons.
Through comparative analysis, researchers gain insights that help in determining which tool best suits their specific needs, thus enhancing the quality of their research. Understanding the strengths and weaknesses of different prediction tools not only aids in optimizing outcomes but also fosters ongoing improvement in the evolution of these technologies.
Strengths of AlphaFold versus Competitors
AlphaFold has carved a niche for itself in the field of protein structure prediction, boasting several advantages that set it apart from its peers:
- Accuracy: AlphaFold’s predictions often exceed those of traditional methods and other contemporary tools in precision. It makes use of deep learning techniques and vast datasets to predict protein conformations with remarkable accuracy, allowing researchers to obtain reliable models rapidly.
- Speed of Prediction: In comparison to some conventional tools that necessitate extensive computational time and resources, AlphaFold can return predictions in a fraction of the time. This efficiency is pivotal for researchers requiring quick insights into protein structures.
- User-friendly Interface: The online platform of AlphaFold is designed to be intuitive. Researchers, even those with limited computational expertise, can navigate its features without significant training.
- Integration of Historical Data: AlphaFold effectively leverages known protein structures and evolutionary information, enhancing the reliability of its predictions. Many existing tools may not harness such extensive historical data efficiently, sometimes leading to inaccurate outputs.
- Open Access: The availability of AlphaFold as a freely accessible tool democratizes access to this powerful technology, allowing scientists from diverse backgrounds to explore structural biology without financial constraints.
Limitations of AlphaFold Compared to Traditional Methods
Despite its groundbreaking capabilities, AlphaFold is not without limitations when placed alongside traditional methods:
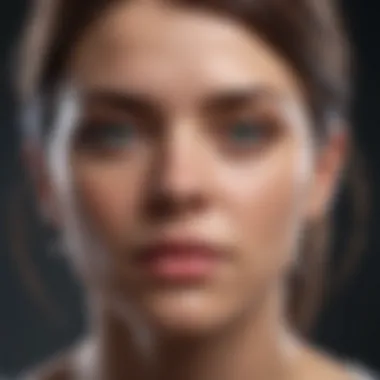
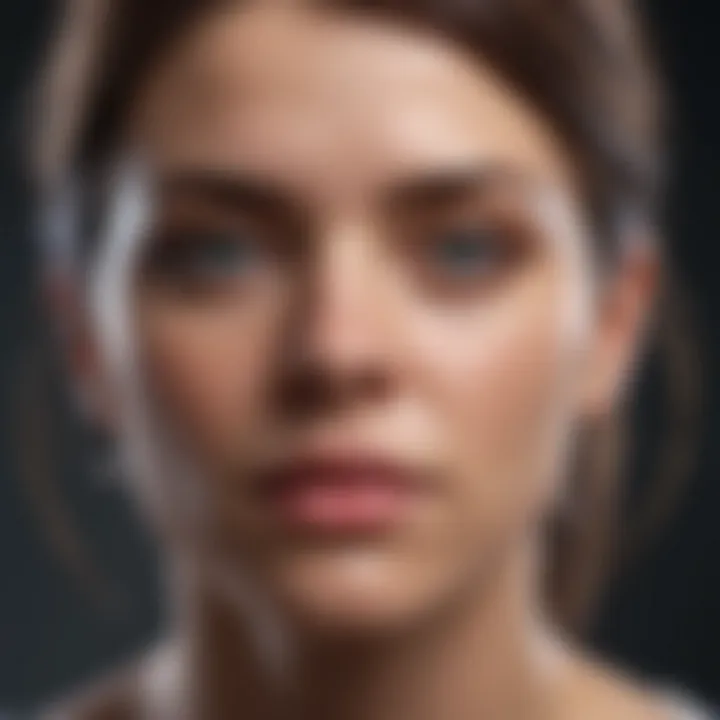
- Range of Application: Traditional methods, such as X-ray crystallography or nuclear magnetic resonance, can obtain structures in conditions that may not be captured by computational predictions. Therefore, for certain proteins, these classic approaches remain indispensable.
- Dependency on Quality of Input Data: AlphaFold’s performance heavily relies on the quality of the protein sequence input. If the data provided is flawed, predictions can result in erroneous models, whereas some traditional methods can still yield results even under challenging conditions.
- Computational Limitations: Although AlphaFold is designed to work efficiently, the need for high-quality GPU resources may not be feasible for every lab, particularly smaller institutions with limited funds.
- Lack of Experimental Validation: AlphaFold's predictions substantiate theoretical models but might not correlate perfectly with experimental results. This disconnect means researchers need to approach findings with a discerning eye, which can shift the focus back to traditional validation techniques.
- Limited Insight into Dynamics: While AlphaFold provides a static picture of proteins, it might not effectively capture dynamic changes that occur during biological processes. In contrast, certain traditional methods excel in revealing functional dynamics of proteins over time.
"In the race for accurate protein prediction, while tools like AlphaFold are making significant strides, the tried-and-true methods still hold considerable weight in experimental validation."
In summary, the comparative analysis of AlphaFold with other prediction tools highlights a mix of revolutionary advantages and traditional necessities. With the right understanding, researchers can leverage both advanced and classic methods concurrently, enhancing their scientific inquiries into intricate biological questions.
Ethical Considerations and Challenges
Understanding the implications of utilizing AlphaFold online goes beyond technical prowess. It treads into the territory of ethics—crucial in an age where AI is becoming integral to scientific research. As AlphaFold pushes the boundaries of what's achievable in protein folding predictions, it also brings to light a set of ethical dilemmas that demand thoughtful reflection from the scientific community. These dilemmas involve maintaining scientific integrity, addressing data biases, and ensuring responsible research practices. In this section, we will explore the significance of these ethical considerations in the context of AlphaFold.
Impact of AI on Scientific Integrity
With the emergence of AI tools like AlphaFold, a worrying trend has surfaced—relying on algorithms for scientific breakthroughs can inadvertently sidestep traditional research methodologies. This shift might lead to a decrease in emphasis on reproducibility and verification that have long been cornerstones of scientific inquiry. By providing rapid insights, AlphaFold can sometimes obscure the meticulous validation process essential for confirming findings.
Here are a few points to consider related to AI’s impact on scientific integrity:
- Over-reliance on Automation: As researchers lean on AI-driven results, a risk exists that the underlying questions may be overlooked in pursuit of quick answers.
- Verification of Results: The pace of discovery, expedited by AlphaFold, requires that researchers adapt methods of corroborating findings. Ensuring predictions from AI models align with experimental results becomes increasingly vital.
- Transparency: The algorithms and datasets utilized must be transparent. Without clear documentation, trust in AI-generated insights may dwindle.
"Ethical practices in AI research aren’t just a moral obligation; they shape the credibility of scientific progress."
Tightening the oversight around AI applications in research fosters a culture of integrity and trust.
Addressing Bias in Data Sets
The efficacy of AlphaFold, much like any AI tool, hinges on the quality of its training data. However, if the data is biased, the outcomes will inherently reflect those biases. This can lead to skewed interpretations of biological processes, especially in underrepresented proteins or complex structures that don't align with mainstream scientific literature.
Here are key points to help navigate the issue of bias in data sets:
- Diverse Training Data: Efforts should be made to ensure that the training datasets encompass a wide range of biological diversity. This includes variations across species and experimental conditions to prevent a narrow view of protein structures.
- Continuous Evaluation: As new data becomes available or new methods of collecting data emerge, the existing datasets should be reassessed and refreshed. Keeping the datasets relevant enhances the reliability of AlphaFold’s predictions.
- Collaborative Efforts: Involving a broader community in the dataset creation process can minimize bias. Input from various research institutes and experts ensures a comprehensive representation of protein characteristics.
Bias is a double-edged sword that can misinform research findings. Addressing these concerns proactively can bolster the credibility of findings derived from AlphaFold's capabilities.
Navigating the ethical landscape surrounding AlphaFold is vital for the continued trust and acceptance of AI tools in the scientific community. Ensuring integrity in research methods and a focus on reducing biases will help pave the way for innovative, yet responsible scientific advancements.
Future Perspectives of AlphaFold
As we look toward the future, the potential of AlphaFold holds immense implications for diverse fields like bioinformatics, drug discovery, and disease modeling. Scientific progress often hinges on our capacity to predict and understand protein structures, and AlphaFold sets a new gold standard in this regard. Its evolving capabilities can foster innovation and enhance research efforts in ways that were previously unimaginable.
Advancements on the Horizon
With rapid advancements in artificial intelligence and computational biology, one can anticipate several exciting enhancements to AlphaFold’s functionalities. These might include:
- Higher Accuracy: Expect improvements in prediction accuracy, thanks to machine learning algorithms that continuously learn from new data sets.
- Complex Protein Structures: Future iterations might be able to model more complex protein arrangements, including multi-chain formations and interactions with other biomolecules.
- Real-time Updates: Integration with cloud-based solutions could enable real-time updates and collaborative research efforts across global teams.
Moreover, extending AlphaFold's applicability to membrane proteins, which are notoriously difficult to predict, could lead to breakthroughs in various critical areas like pharmacology and signal transduction. This will not only aid researchers in understanding cellular mechanisms better but also allow for more effective drug development strategies.
Integration into the Scientific Community
The transition of AlphaFold from a pioneering tool to a staple resource in the scientific community is essential. This transition is facilitated through:
- Collaborative Platforms: Increasingly, online platforms can be developed to house AlphaFold’s outputs, allowing researchers to share their findings, hypotheses, and datasets.
- Training Programs: Educational institutions could incorporate training around AlphaFold into their curriculums, ensuring that the next generation of researchers is adept at using and building upon this tool.
- Interdisciplinary Research: Promoting collaborative projects across disciplines, such as genetics, biochemistry, and computational sciences, could lead to surprising discoveries and innovative applications.
Adopting AlphaFold into the scientific community enhances both the breadth and depth of research possibilities while grounding them in robust scientific knowledge. Ultimately, as AlphaFold matures, it can solidify its role as a vital part of contemporary scientific inquiry, bridging gaps and catalyzing groundbreaking discoveries.
Ending
As we wrap our exploration into the world of AlphaFold, it becomes apparent just how transformative this tool is for the fields of biology and bioinformatics. The significance of AlphaFold extends beyond mere protein structure prediction; it plays a pivotal role in addressing some of the most complex challenges in modern science. The intricate dance of amino acids folding into functional shapes is no longer a mystery relegated to speculation.
Summing Up AlphaFold's Impact
The impact of AlphaFold is far-reaching, influencing various domains of research and application. Here are some crucial points that underline its significance:
- Revolutionizing Structural Biology: With its ability to accurately predict protein structures, AlphaFold opens the door to a deeper understanding of biological processes and mechanisms.
- Accelerating Drug Discovery: The precision of AlphaFold's predictions aids in the identification of potential drug targets. This can streamline the lengthy drug development process.
- Facilitating Collaborative Research: The online accessibility invites collaboration among researchers, allowing diverse scientific minds to leverage its capabilities and share insights.
- Enhancing Educational Opportunities: Students and educators can utilize AlphaFold as a learning tool, enriching their curriculum with real-world applications and cutting-edge technology.
"AlphaFold not only predicts protein structures but also reshapes the landscape of biological research, offering tools and insights previously thought unattainable."
While the tool has its limitations, especially when compared to traditional methods, its contribution cannot be overstated. The insights gained from using AlphaFold will continue to refine our understanding of biology and pave the way for future advancements in healthcare and science. As researchers and practitioners, embracing AlphaFold's capabilities can lead to transformative changes in how we approach protein science and beyond.