Navigating FDA Regulations in the Age of AI
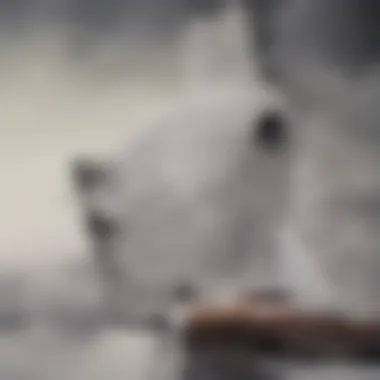
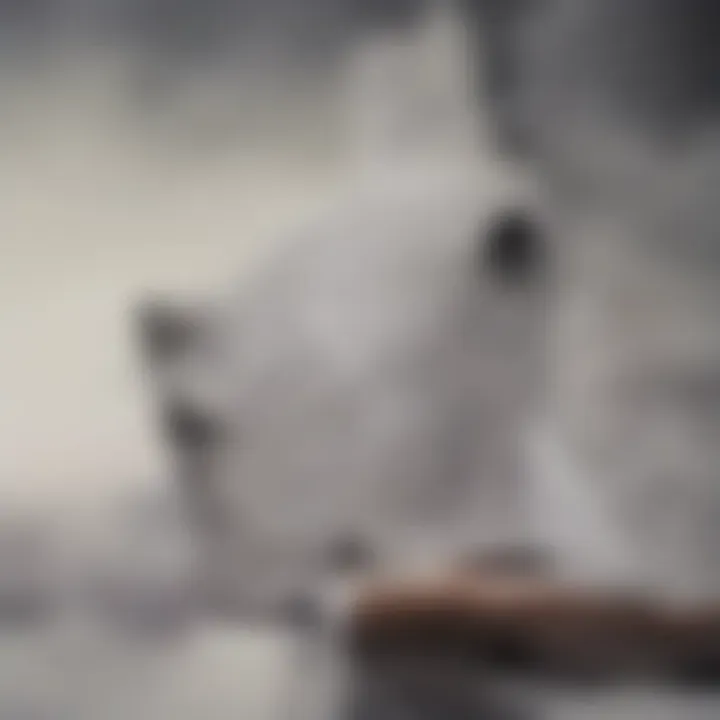
Intro
As artificial intelligence (AI) technologies advance, their application in healthcare becomes increasingly prevalent. The U.S. Food and Drug Administration (FDA) plays a crucial role in ensuring that these technologies are safe and effective. This intertwining of AI and regulatory oversight is complex, requiring a nuanced understanding of both the technology and the regulatory frameworks that govern it. Exploring this interplay is essential not only for the current landscape of medical devices and therapeutics but also for future advancements in the sector.
AI has the potential to transform various aspects of healthcare, including diagnosis, treatment planning, and patient monitoring. However, this transformation comes with challenges. The FDA, tasked with the oversight of medical devices, must adapt its regulatory processes to account for the unique features and risks of AI technologies. These include machine learning algorithms that can evolve over time, making traditional static testing methods less effective.
The article will address how existing FDA regulations align—or conflict—with the rapid advancements in AI. It will also examine the ethical implications that arise from the integration of AI in medical practices. The discussion will highlight current challenges, emphasize regulatory compliance, and showcase pathways for improved collaboration between AI innovators and regulatory bodies.
This exploration aims to provide a rich, detailed narrative for students, researchers, educators, and professionals, clarifying the significance of understanding the relationship between AI and FDA regulations in shaping the future of healthcare.
Understanding the FDA's Role
The role of the U.S. Food and Drug Administration (FDA) in regulating artificial intelligence (AI) technologies in healthcare is critical. This section provides insights into how the FDA ensures safety and efficacy as it relates to advancing AI applications. Given the rapid progression of AI in medical settings, understanding the FDA's function is essential for stakeholders navigating the complex landscape of healthcare innovation.
Overview of the FDA
The FDA is responsible for protecting public health by ensuring the safety and efficacy of drugs, biological products, medical devices, and food supplies. Established under the Federal Food, Drug, and Cosmetic Act, the agency has a long history of regulatory oversight. This background gives the FDA a robust platform to evaluate new technological advancements, including AI. With AI increasingly influencing healthcare, the FDA's establishment of clear guidelines has become even more important. Understanding the history of the FDA helps provide context for its regulatory approaches and decision-making processes.
FDA's Mission and Vision
The FDA's mission is to protect public health by ensuring the safety, efficacy, and security of human and veterinary drugs, biological products, and medical devices. Vision-wise, it aims to support innovation while maintaining rigorous safety standards. In relation to AI, this balance is particularly crucial. Innovations in AI can lead to significant advances in diagnostics and patient care, but they may also introduce potential risks. The FDA envisions a future where safe AI technologies can function seamlessly alongside existing medical devices, enhancing patient outcomes.
Regulatory Responsibilities
The regulatory responsibilities of the FDA include evaluating new medical products, issuing guidance documents, and monitoring the compliance of marketed products. For AI, the FDA adopts a risk-based approach to classification, categorizing products based on their potential impact on patient health. This classification affects the level of regulatory scrutiny each AI technology will face. The FDA also collaborates with industry stakeholders to establish best practices and facilitate discussions about technological advances. Keeping up with these responsibilities is crucial for developers and researchers working in AI healthcare.
"The FDA's role is not just about approvals; it's about ensuring that innovations genuinely benefit patients without jeopardizing their safety."
In summary, understanding the FDA's role is vital for navigating the intersection of AI and healthcare. As AI technologies evolve, the FDA's mission and responsibilities will continue to shape the landscape of medical innovation. This will be further explored in subsections dedicated to specific AI applications in healthcare.
Artificial Intelligence in Healthcare
Artificial Intelligence (AI) is transforming the healthcare sector in meaningful ways. Its importance lies not just in improving efficiency, but also in enhancing outcomes for patients and healthcare providers. As this article explores, the interplay between AI and FDA regulations is crucial for fostering innovation while ensuring safety and efficacy. Understanding AI's role in healthcare involves examining how it can assist in diagnostics, treatment recommendations, and even operational efficiencies.
The Rise of AI Technologies
The development of AI technologies has been rapid in recent years. Advancements in machine learning and deep learning have created systems that can analyze vast amounts of medical data. These technologies provide higher accuracy in diagnosing diseases compared to traditional methods. Tools like IBM Watson and Google DeepMind have made headlines for their capabilities in predicting patient outcomes and identifying patterns in large datasets. These innovations represent a paradigm shift in how healthcare operates, making treatment more personalized and data-driven.
Applications of AI in Medicine
AI applications in medicine are numerous and varied. Here are some key areas where AI is making a significant impact:
- Diagnostic Imaging: AI algorithms can interpret images from X-rays, MRIs, and CT scans with precision. They assist radiologists by highlighting anomalies and ensuring no critical details are overlooked.
- Clinical Decision Support: Systems powered by AI provide healthcare professionals with data-driven insights, improving decision-making processes in pathology, pharmacology, and treatment protocols.
- Predictive Analytics: AI can predict outbreaks, patient admissions, and interventions needed. For instance, algorithms can analyze historical data to foresee trends in illnesses in specific communities.
The applicability of AI in these domains showcases its potential to revolutionize everyday practices in healthcare.
Impact on Patient Care
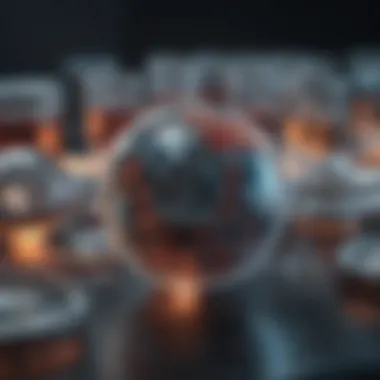
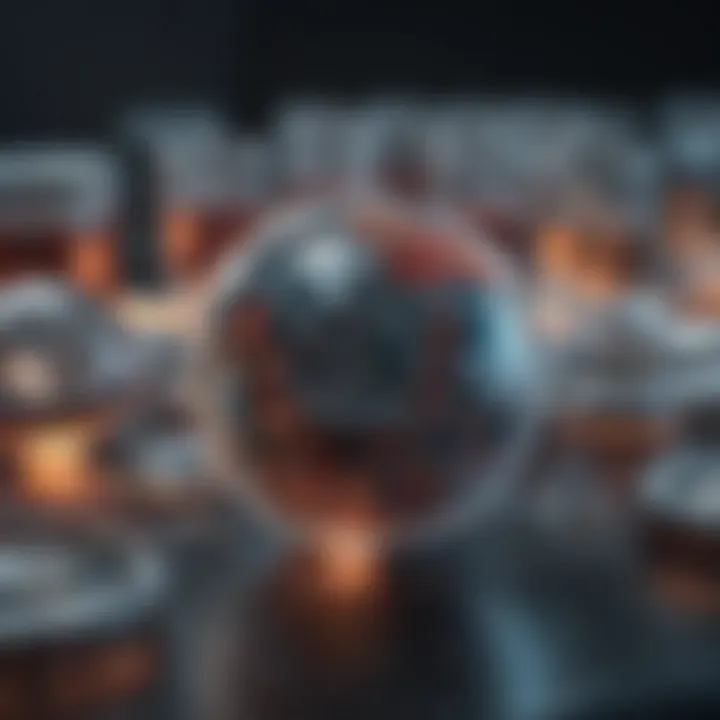
The integration of AI into patient care can lead to tangible improvements. Here are several implications for patient care:
- Enhanced Accuracy: With AI's analytical capabilities, misdiagnoses and treatment errors can be significantly reduced.
- Faster Results: AI-driven tools can process information much more quickly than human counterparts. This speed allows for faster treatment, which can be critical in emergency situations.
- Personalized Treatment Plans: AI systems analyze patient data to recommend customized treatment options, taking into account a patient’s unique history, genetics, and lifestyle.
"The intersection of AI and healthcare not only improves efficiency but also transforms the patient experience, making care more individualized and accessible."
In summary, AI in healthcare is not just a technological advancement; it is a crucial development that reshapes the landscape of patient care, ensuring better outcomes and enhancing the overall system.
Navigating FDA Regulations for AI
Navigating FDA regulations for Artificial Intelligence is crucial in ensuring that AI technologies comply with established safety and efficacy standards in healthcare. A clear understanding of these regulations not only facilitates smoother approval processes but also fosters innovation that aligns with public health interests. The intricacies of the regulatory landscape can pose significant hurdles for developers. Adequate navigation can lead to the successful introduction of intelligent systems that enhance patient care and streamline healthcare operations. In this context, it becomes necessary to explore the challenges within regulatory frameworks, current guidelines for AI approval, and real-world examples of recent approved AI systems.
Challenges in Regulatory Frameworks
The regulatory frameworks governing AI in healthcare face multiple challenges. One significant issue is the rapid evolution of AI technologies outpacing existing regulations. Traditional frameworks, primarily designed for conventional medical devices, often do not account for the dynamic nature of AI systems, which can learn and adapt over time. This creates ambiguity regarding the extent of evaluation required prior to market entry.
Moreover, there is a lack of standardized guidelines tailored specifically for AI applications. Different types of AI models (like supervised, unsupervised, and reinforcement learning) present varied risks, complicating risk assessment.
"A standardized approach is needed to ensure that regulatory processes keep pace with AI advancements, providing clarity for both developers and regulators."
Adding to the complexity, there is a need for clarity surrounding data usage and patient privacy. The AI models often rely on large datasets for training, raising questions about consent and data protection. Addressing these issues necessitates a collaborative approach involving AI developers, regulatory agencies, and other stakeholders.
Current Guidelines for AI Approval
Current guidelines for AI approval by the FDA establish a framework aimed at integrating these technologies into the healthcare ecosystem while prioritizing safety. In 2021, the FDA published a discussion paper outlining a proposed regulatory pathway specifically for AI and machine learning-based software as a medical device. This document emphasizes a need to assess not only the effectiveness but also the continual performance throughout the lifecycle of the product.
Key highlights of the current guidelines include:
- Pre-Market Submission: Submissions must provide validation to prove that the AI system works consistently across various scenarios.
- Post-Market Surveillance: Technologies must include mechanisms for real-world performance monitoring to ensure ongoing efficacy and safety.
- Transparency Requirements: Developers are encouraged to provide understandable explanations of algorithms to promote trust among clinicians and patients.
While these guidelines mark a positive step, there is ongoing debate about the applicability and comprehensiveness of such measures.
Case Studies of Recent Approvals
Examples of recent FDA approvals illustrate progress in navigating regulations for AI technologies. One prominent case is the approval of IDx-DR, an AI system designed for autonomous detection of diabetic retinopathy. This system uses deep learning algorithms to analyze retinal images and provide diagnostic recommendations without requiring clinician interpretation.
Another significant case includes the FDA's approval of AI-based software like Lunit INSIGHT, which aids radiologists by enhancing detection rates of lung cancer in chest X-rays. The success of these systems demonstrates that when AI products adhere to outlined regulatory guidelines, they can safely enhance diagnostic accuracy and efficiency.
These case studies reflect the potential for AI to transform healthcare when regulatory frameworks adapt and evolve to accommodate their unique characteristics.
Ethical Considerations in AI and FDA
The integration of Artificial Intelligence in healthcare brings forth critical ethical considerations that must be acknowledged in conjunction with FDA regulations. As AI technologies advance, they fundamentally alter how patient care is delivered. Hence, ethical frameworks shape the way AI systems should be designed, implemented, and governed. These frameworks ensure that the deployment of AI not only adheres to federal regulations but also upholds values that promote patient safety, equity, and transparency.
Ethical Frameworks for AI Use
Establishing ethical frameworks is essential in guiding the use of AI technologies within the healthcare context. Such frameworks outline principles that serve as a foundation for decision-making processes involving AI. Key components include
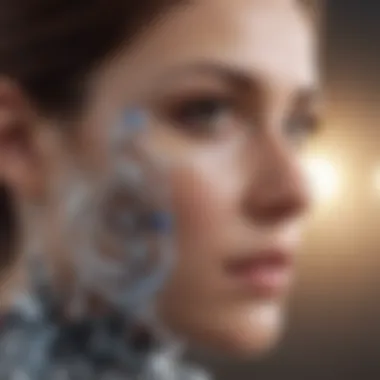

- Accountability: Developers of AI must take responsibility for their creations. This includes understanding potential risks and ensuring that appropriate measures are in place to mitigate harm.
- Transparency: The operations of AI algorithms should be clear, allowing stakeholders to understand how decisions are made. Openness in data usage and model functioning is crucial to gain trust.
- Fairness: AI applications must strive to minimize biases that could result in unequal treatment among diverse patient populations.
Frameworks like these ensure that innovations introduce net benefits to society while protecting individual rights. Such guidelines encourage ethical reflection and deliberation around complex issues that arise in healthcare technology.
Bias and Fairness in AI Algorithms
Bias in AI algorithms represents a significant concern that can lead to unfair treatment and health disparities. AI systems learn from data, which may inherently contain biases reflecting historical inequalities. Thus, if not addressed, an AI system can perpetuate or even exacerbate these biases.
Some considerations include:
- Data Quality: Ensuring that data used to train AI models is comprehensive and representative of the entire population. This reduces the chances of systemic bias.
- Algorithmic Audits: Regular assessments of algorithms can help identify biases and correct them before they translate into practical applications.
- Diverse Development Teams: Including individuals from varying backgrounds in AI development teams can provide diverse perspectives, helping to illuminate blind spots.
Addressing these issues not only enhances the fairness of AI systems, but it also fosters an equitable healthcare environment wherein all individuals receive appropriate care.
Patient Privacy and Data Protection
In the realm of healthcare, patient privacy is paramount. The utilization of AI often involves processing large volumes of sensitive data, raising important questions about data protection methods. Regulatory bodies, including the FDA, impose strict guidelines to ensure patient data is handled with care.
Key aspects of patient privacy include:
- Informed Consent: Patients must be educated about how their data will be used, allowing them to make informed decisions about participation.
- Data Anonymization: HL7 and FHIR formats can help in anonymizing data, reducing risks of identification while still allowing for valuable analysis.
- Compliance with Regulations: Adhering to laws such as HIPAA is essential for any AI system operating within healthcare, mandating rigorous standards for data handling.
Maintaining patient privacy not only safeguards individuals but also encourages further trust in AI technologies that can lead to innovative healthcare solutions.
Future Directions in AI Regulation
Emerging Technologies and Their Impact
Emerging technologies, such as machine learning and neural networks, are changing the landscape of healthcare. These technologies enable systems to learn from data, creating diagnostic tools that can outperform human capabilities in certain areas. For example, AI can analyze medical images more precisely than some human radiologists. This potential can lead to earlier detection of diseases, including cancer.
Laudably, the FDA's framework is adapting to these changes. The Agency has initiated efforts to create a more flexible regulatory process, recognizing the need to accommodate ongoing updates to AI systems.
"The fast rate of AI development necessitates regulation that can keep pace without stifling innovation."
The Role of Public Health Policy
Public health policy plays an essential role in guiding the safe deployment of AI technologies. With the increasing integration of AI in healthcare, policies must ensure that these innovations improve health equity. Lack of regulatory clarity can lead to disparities in access to beneficial technologies. Government agencies and policymakers must collaborate closely with the FDA to align public health objectives with regulatory frameworks. This ensures that AI advancements serve the needs of all population segments.
Effective public health policies also involve stakeholder engagement. Input from healthcare professionals, patient advocates, and technology developers can lead to more comprehensive and inclusive regulations. This collaborative approach can mitigate risks related to safety and efficacy while ensuring that innovations are accessible.
Collaborative Approaches for Innovation
To address the challenges of regulating AI in healthcare, collaborative approaches are necessary. Partnerships between the FDA, tech companies, and healthcare providers can foster an environment of shared knowledge. Such collaboration can result in the development of best practices for the integration of AI into healthcare.
Encouraging stakeholders to work together allows for the sharing of data and insights, which can benefit the entire system. Moreover, piloting new technologies in controlled environments can provide valuable feedback before wider implementation.
Key Benefits of Collaborative Approaches:
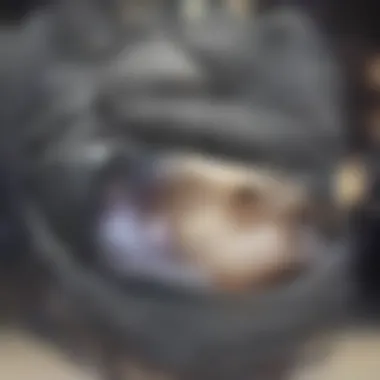
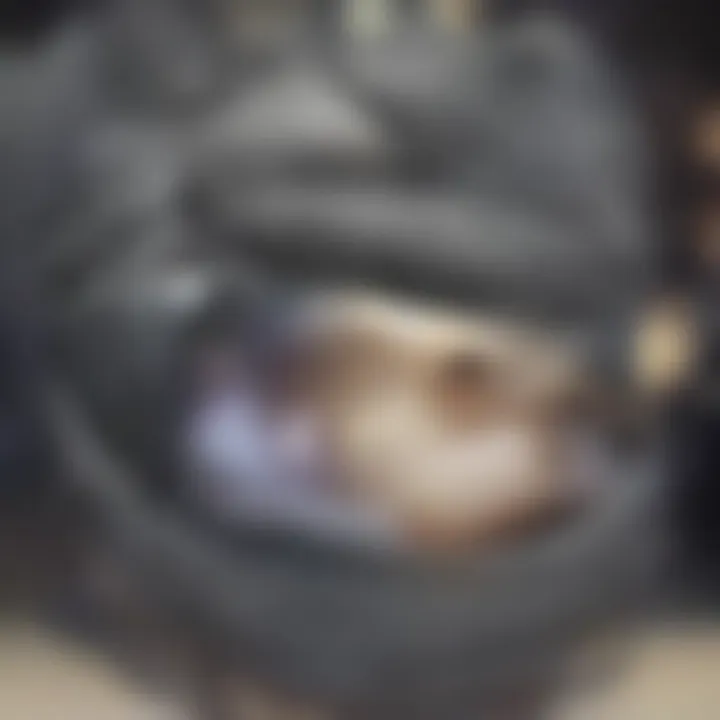
- Improved understanding of emerging technologies.
- Enhanced regulatory frameworks informed by real-world applications.
- Faster identification of potential risks and challenges.
Navigating the AI Ecosystem
Navigating the AI ecosystem is crucial in understanding how artificial intelligence interfaces with regulatory frameworks, especially those established by the FDA. The interplay within this ecosystem can determine the success or failure of AI innovations in healthcare. Key elements include the stakeholders involved, investment trends, and the importance of building effective partnerships. Each of these components plays a vital role in shaping the future of AI in the medical field.
Stakeholders in AI Development
The AI development landscape in healthcare comprises various stakeholders, including technology companies, healthcare providers, regulatory bodies, and patients. Each group has unique contributions and expectations.
- Technology Companies: These are primarily responsible for developing AI solutions. Their goal is to create effective, efficient, and user-friendly technologies, while facing the challenge of meeting regulatory requirements.
- Healthcare Providers: They will utilize AI in patient care. Their feedback is essential for refining algorithms and ensuring practical application in clinical settings.
- Regulatory Bodies: Agencies like the FDA serve a gatekeeping role, evaluating AI tools for safety and efficacy before they can reach the market. Their guidelines inform both developers and providers on what is acceptable.
- Patients: They are also stakeholders, as they stand to benefit from AI advancements in healthcare but must also have their privacy and data protections safeguarded.
Engagement between these stakeholders fosters an ecosystem that can effectively support and adapt to innovations in AI.
Investment Trends in AI Healthcare Solutions
The healthcare sector has witnessed notable investment trends in the realm of AI technology. This investment landscape is characterized by various factors that influence the availability and development of resources.
- Venture Capital Funding: There has been a surge in venture capital flowing into AI healthcare startups. This reflects confidence in AI's potential to transform healthcare delivery and outcomes.
- Corporate Partnerships: As established companies recognize the potential of AI, they seek out partnerships with startups. These collaborations often stimulate innovation and speed up the development process.
- Government Grants and Funding Programs: Many governments are investing in AI research within healthcare, aiming to foster breakthroughs that can improve public health.
Understanding these trends allows stakeholders to better navigate the financial aspects of AI development, ensuring that innovative solutions can be effectively funded and brought to market.
Building Effective Partnerships
The success of AI-driven healthcare solutions heavily relies on building effective partnerships across different stakeholders.
- Public-Private Collaborations: These partnerships can facilitate knowledge sharing and resource allocation. They can help lead to standards that ensure safety while promoting innovation.
- Academic Partnerships: Engaging with universities and research institutions can provide access to cutting-edge research and technological advancements. This bridges the gap between theory and practice in AI application.
- Cross-Industry Alliances: AI’s nature often requires interdisciplinary approaches. For instance, partnerships between tech entities and regulatory bodies can help to create a more standardized understanding of compliance and deployment strategies.
To create a robust AI ecosystem in healthcare, it is essential to prioritize synergy between these collaborative efforts. This approach not only enhances innovation but also addresses regulatory complexities that can hinder progress.
"The integration of AI in healthcare demands a concerted effort from all stakeholders to ensure that advancements align with regulatory expectations and healthcare needs."
In summary, navigating the AI ecosystem is a multifaceted endeavor that requires a comprehensive understanding of different stakeholders, investment trends, and partnership dynamics. The interplay between these elements is pivotal to advancing how AI can effectively and safely transform healthcare.
The End and Key Takeaways
The intersection of FDA regulations and Artificial Intelligence is a crucial area in healthcare. Understanding this complex relationship is essential for various stakeholders, including healthcare professionals, AI developers, and regulatory bodies. The implications of integrating AI in medical practice extend beyond technology; they also encompass ethical standards and patient safety. Exploring these elements provides clarity on the evolving landscape of healthcare.
Summarizing Findings
The article highlights several significant points regarding the interplay between FDA regulation and AI. Firstly, the FDA's role is not merely administrative; it actively shapes the deployment of AI technologies in healthcare. Current guidelines reflect specific challenges related to the rapid advancement of AI. The necessity for a clear regulatory framework is evident, especially as AI applications continue to expand. There is a strong emphasis on ensuring that AI tools are safe, effective, and equitable for patients.
Moreover, ethical considerations, such as bias in AI algorithms and data privacy, cannot be overlooked. It becomes apparent that stakeholders must work closely together to address these concerns while innovating medical practices.
Implications for the Future
The future of healthcare will likely see AI becoming even more integrated within medical systems. However, this integration must occur within a robust regulatory framework. As AI technologies evolve, the FDA's guidelines will also need to adapt. This dynamic nature of regulatory frameworks can lead to innovative medical devices and treatments that enhance patient outcomes. The collaborative efforts among the FDA, healthcare providers, and AI developers will play a central role in defining the success of AI integration.
In summary, stakeholders should expect to face obstacles but also promising opportunities. The trajectory of AI in healthcare will depend on navigating these challenges while prioritizing patient safety and ethical standards.
Recommendations for Stakeholders
To effectively engage with the evolving landscape of AI in healthcare, stakeholders should consider several recommendations:
- Stay Informed: Continuous education on the latest FDA guidelines is essential for developers and healthcare providers alike.
- Foster Collaboration: Engage in partnerships with regulatory agencies, industry leaders, and academia. Collaborative efforts can lead to more effective regulatory processes and innovative solutions.
- Prioritize Ethical Standards: AI developers must focus on eliminating bias and ensuring data privacy in their algorithms.
- Advocate for Clear Regulations: Stakeholders should push for clear, adaptable regulatory guidelines that can keep pace with technological advancements.
- Monitor Patient Outcomes: Implementing AI tools should come with ongoing assessments of their impact on patient care, ensuring that the technology serves as a benefit rather than a risk.