Global Forecast: Interdisciplinary Insights and Projections
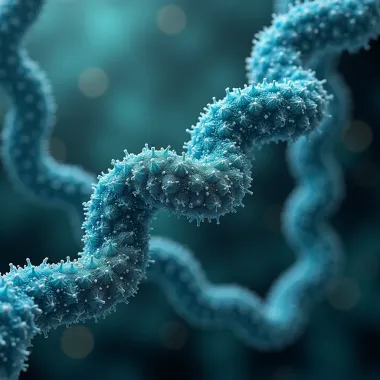
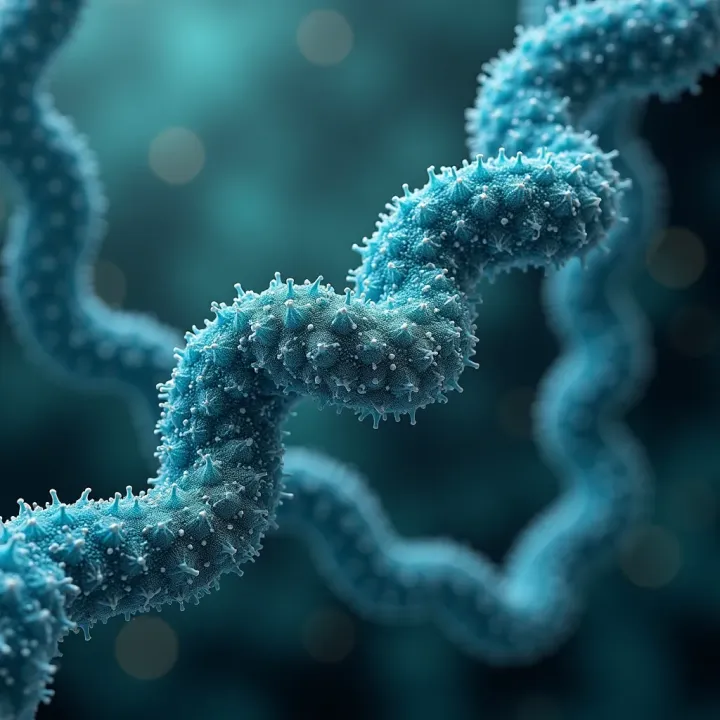
Intro
Global forecasting has emerged as an essential element in various scientific disciplines. It encapsulates the methodologies and tools that researchers use to anticipate future trends and behaviors. Understanding these forecasting techniques can illuminate pathways for informed decision-making in agriculture, environmental policy, healthcare, and more. Academia, industry, and government are increasingly recognizing the need for evidence-based projections to navigate complexities of the modern world.
This article will explore diverse methods and frameworks used in global forecasting, examining how different fields like biology, chemistry, and physics utilize these methodologies. By integrating interdisciplinary knowledge, we can draw richer insights into potential future scenarios. Moreover, we aim to provide understanding of the implications these forecasts have on policy and societal adaptation.
Methodologies
Description of Research Techniques
In global forecasting, various research techniques come into play. Quantitative approaches dominate the field, especially where numerical data is available. Statistical modeling, including time series analysis and regression modeling, helps in making predictions based on existing data sets. For instance, climate scientists use models that simulate atmospheric conditions to predict future weather patterns.
Furthermore, qualitative techniques such as Delphi methods and expert surveys can bring multi-faceted perspectives. They rely on the insights from specialists in respective fields. These techniques help in understanding complex systems where numerical data alone might fall short. Combining quantitative and qualitative methods creates a balanced approach to forecasting.
Tools and Technologies Used
Technological advancement has transformed the landscape of global forecasting. Researchers now employ sophisticated software tools that enhance data analysis capabilities.
Some popular tools include:
- MATLAB: Utilized for complex mathematical modeling and simulation.
- R: A programming language widely used for statistical computing and graphics.
- Python: Increasingly popular for data manipulation and machine learning applications.
Additionally, Geographic Information Systems (GIS) are crucial for environmental forecasting, allowing visualization and analysis of spatial data.
"Predictive science transcends the boundaries of individual disciplines, enriching our understanding of global trends through collaboration."
Discussion
Comparison with Previous Research
Historically, forecasting relied heavily on linear models, which often provided simplistic views of complex interactions in nature and society. Recent studies illustrate a shift to more dynamic and adaptive models that account for variability over time. For instance, ecosystem modeling has advanced, moving beyond static representations to include feedback loops and nonlinear dynamics, as observed in works from the past decade.
Theoretical Implications
The implications of adopting interdisciplinary forecasting methods are significant. They challenge conventional theoretical frameworks and necessitate a reevaluation of existing hypotheses. As researchers from various domains collaborate, the boundaries of traditional theories expand, leading to richer conceptual understandings of phenomena.
Overall, the interconnectedness of scientific disciplines in forecasting enhances our capability to address and resolve pressing global issues.
Foreword to Global Forecasting
Global forecasting plays a critical role in understanding the present and making informed decisions for the future. By synthesizing data across various disciplines, forecasting enables us to anticipate trends, assess risks, and allocate resources accordingly. In an interconnected world facing challenges such as climate change, health crises, and economic shifts, the ability to predict future scenarios becomes crucial.
Definition and Importance
Forecasting refers to the use of quantitative and qualitative analyses to estimate future conditions or events. This process is essential in various fields such as economics, biology, and social sciences. The importance of forecasting lies in its capacity to aid in decision-making. By analyzing patterns and trends, professionals can strategize effectively, whether in business or public policy. Additionally, forecasting enhances preparedness for potential adverse events, allowing societies to navigate uncertainties with more confidence. The predictive models developed can influence resource management, investment strategies, and even societal responses to emergent situations.
Historical Context
The practice of forecasting is not new. Historical records show that societies have always relied on observational data to anticipate seasonal changes or agricultural yields. Ancient civilizations employed various methods to predict weather and natural phenomena. Over the centuries, the advent of the scientific method allowed for more systematic approaches, leading to major advancements in statistical techniques in the 20th century. This progression laid the groundwork for the sophisticated forecasting models we utilize today. The evolution of technology has also facilitated access to vast amounts of data, giving rise to more complex predictive strategies that drive contemporary forecasting.
"Forecasting serves as a bridge between historical data and future possibilities, enabling nuanced understanding and action."
Methodologies in Forecasting
Understanding the methodologies in forecasting is essential. These methodologies shape how data is interpreted and predictions are made across various disciplines. They allow researchers and practitioners to translate complex data into meaningful insights. This facilitates informed decision-making in areas such as public health, environmental science, and economics. Each methodology has its unique strengths and limitations that must be carefully weighed to achieve accurate outcomes.
Quantitative vs Qualitative Approaches
Forecasting methodologies can be broadly categorized into quantitative and qualitative approaches. Quantitative approaches rely heavily on numerical data and statistical analysis. They employ mathematical models to understand patterns and predict future events. Common methods include regression analysis, time series analysis, and econometric modeling. They are particularly useful when extensive historical data is available. Quantitative methods often lead to more precise predictions.
On the other hand, qualitative approaches focus on insights and subjective assessments. These methods might involve expert opinions, case studies, and surveys. They are invaluable in scenarios where numerical data is scarce or new phenomena are being studied. For example, predicting public response to a new policy may require qualitative analysis, as data may not yet exist. While qualitative methods may lack the predictive power of their quantitative counterparts, they provide context and deeper understanding.
Statistical Models and Techniques
Statistical models form the backbone of quantitative forecasting. They enable analysts to explore relationships between variables and draw inferences. Commonly used statistical techniques include Bayesian models, ARIMA (AutoRegressive Integrated Moving Average), and regression analysis. Each technique has a specific application depending on the context. Bayes' theorem is used widely in risk assessment, while ARIMA is suited for time-series forecasting.
Statistical techniques benefit significantly from historical data. However, they also require assumptions about the data distribution and underlying processes. The accuracy of these models can be compromised if the assumptions do not hold in practice. Thus, understanding the underlying power and constraints of these methods is vital for effective forecasting.
Machine Learning Applications
Machine learning is transforming methodologies in forecasting. Traditional statistical methods often struggle with high-dimensional data or complex relationships. Machine learning algorithms excel in these areas by identifying patterns that may not be immediately visible. Techniques such as neural networks, decision trees, and support vector machines have gained popularity across various fields.
For instance, in public health, machine learning has been used to predict disease outbreaks with impressive accuracy. In finance, it helps investors gauge market trends. However, machine learning models generally require significant amounts of data to train effectively, and they can be more opaque than traditional models. Interpreting their results can be difficult, leading to potential challenges in credibility and transparency.
The selection of the right forecasting methodology is crucial. It directly impacts the quality of predictions and the decisions that follow.
Forecasting in Biology
Forecasting in biology serves as a pivotal area of focus within global forecasting. This branch of study combines a variety of methodologies to predict ecological patterns, species behaviors, and disease outbreaks. With advances in analytical techniques, biologists can enhance conservation efforts, manage resources, and prepare for health crises. The implications are far-reaching not just within the sciences, but also for policy making and public health decisions. By understanding biological forecasting, one can appreciate the interconnections between living organisms and their environments, leading to better outcomes for biodiversity and human health.
Ecosystem Predictions
Ecosystem predictions represent a critical component of biological forecasting. These predictions aim to provide insights into how ecosystems will respond to environmental changes. Factors such as climate change, habitat loss, and species invasions significantly impact these ecological systems. Understanding these dynamics can help inform conservation strategies and ecological restoration.
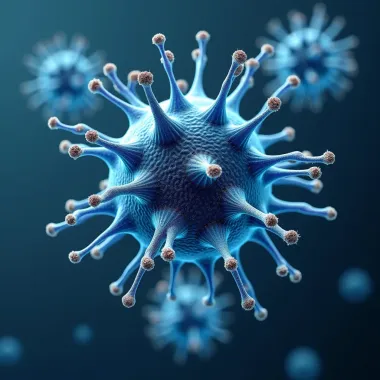
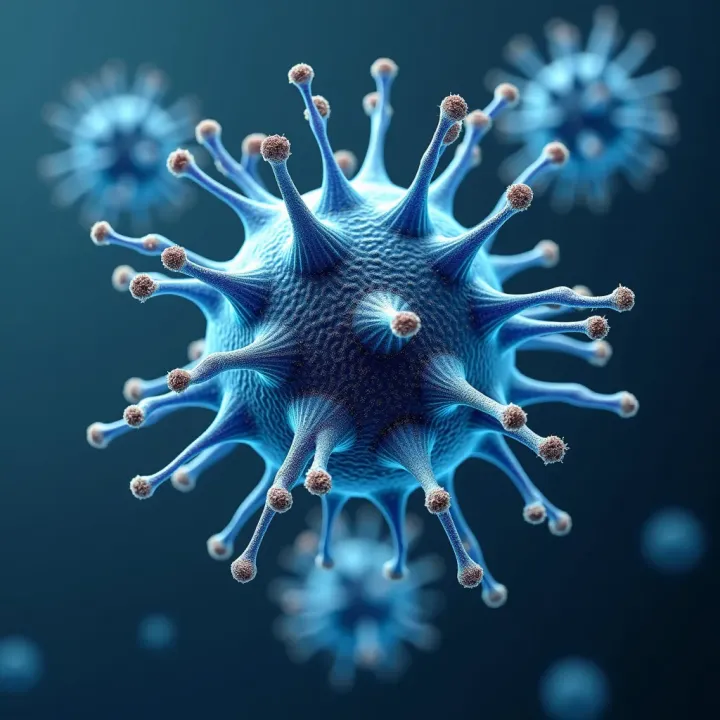
Researchers utilize various models that incorporate data from multiple sources, including satellite imagery, climate data, and species distribution records. By leveraging this information, predictions can illustrate potential shifts in species populations and community structures.
- Benefits of Ecosystem Predictions
- Resource Management: Tools like ecosystems models help in managing natural resources effectively.
- Conservation Strategies: Targeted approaches can focus on preserving vulnerable habitats and species.
- Planning for Change: Anticipating ecosystem shifts aids in developing adaptive management practices.
Moreover, as noted by ecological scientists, "The predictive landscape is not about asserting what is to come, but understanding the probabilities of various outcomes that can unfold." This nuanced approach speaks to the essence of forecasting in ecology, recognizing that uncertainty is a natural part of biological systems.
Disease Outbreak Models
Disease outbreak modeling encompasses another crucial element of forecasting in biology. These models assess how infectious diseases spread and evolve over time. The insights gained are invaluable in preparing for and managing public health crises. Significant events, such as the COVID-19 pandemic, have highlighted the necessity of robust predictive modeling in biology.
Modeling approaches typically include epidemiological models, which can project future cases based on current infections and human behaviors. Key models utilize parameters like transmission rates and mitigation strategies to estimate potential outbreaks.
Key aspects include:
- Contact Patterns: Understanding how human behaviors affect disease transmission.
- Vaccination Impact: Predicting how vaccination rates influence disease spread.
- Environmental Factors: Considering how environmental changes can lead to new outbreaks.
Integrating data from diverse fields, such as genomics, public health, and sociology, enhances modeling's accuracy. > "A well-informed prediction can be the difference between containment and widespread outbreak," emphasizes public health specialists.
Through comprehensive understanding and thoughtful predictions, stakeholders can implement timely measures to safeguard public health. Overall, forecasting in biology reflects the dynamic relationship between organisms and their environments, providing essential tools for anticipation and proactive management.
Forecasting in Chemistry
Forecasting in chemistry holds significant importance as it intersects various scientific fields and offers insights into numerous applications. This section will explore how predictions enhance our understanding of chemical reactions and materials science. By employing advanced methodologies, chemists can not only anticipate outcomes but also innovate solutions crucial for industry and health.
Chemical Reaction Predictions
Chemical reaction predictions are paramount for both academic research and industrial applications. Understanding the rate and product of chemical processes can lead to better efficiency in chemical manufacturing, energy production, and environmental management. For instance, predicting how a substance will react under specific conditions allows chemists to design safer processes.
One significant benefit of these predictions is reducing costs and time. Industries can avoid unnecessary experiments and optimize processes through simulations and predictive models. This streamlining is particularly evident in pharmaceuticals, where knowing the outcomes of reactions can expedite drug development.
Key techniques used include:
- Computational chemistry, which employs quantum mechanics to model reactions.
- Kinetic Models, predicting reaction rates based on reactant concentrations and temperature.
- Machine Learning, enabling the analysis of vast datasets to discover patterns in reaction outcomes.
"The predictability of chemical reactions not only enhances efficiency but also supports innovation across sectors."
Material Sciences Innovations
In the realm of material sciences, forecasting is essential for the development of new materials with desired properties. This field utilizes predictions to guide the synthesis of polymers, composites, and nanomaterials. By understanding how materials behave under various conditions, scientists can create products that meet specific needs, such as increased strength, reduced weight, or improved conductivity.
Moreover, forecasting in this area helps identify potential applications before materials are even synthesized. For example, a newly predicted material may have promising characteristics for use in electronics, batteries, or construction.
Important aspects of material science innovations include:
- Property Prediction: Estimating the mechanical, thermal, and electrical properties of materials.
- Sustainability: Identifying eco-friendly materials to meet regulatory standards and reduce environmental impact.
- Performance Analysis: Understanding how materials perform over time or under extreme conditions.
The interplay of forecasting and innovation not only drives scientific discovery but also feeds into economies by improving product quality and reducing waste.
In summary, forecasting in chemistry is vital for advancing our understanding of chemical processes and material development, fostering innovation while addressing practical challenges in the industry.
Forecasting in Physics
Forecasting in physics plays a critical role in helping us understand and predict phenomena that govern the universe. The precision and accuracy of physical predictions can significantly shape scientific inquiry, technological advancement, and policy formulation. The predictive capabilities of physics allow us to model complex systems, evaluate risks, and design innovative solutions against various societal challenges.
The realm of physics encompasses a wide range of topics, from classical mechanics to modern quantum theories. Each area contributes uniquely to our ability to forecast future events or trends. Most importantly, the insights obtained from these forecasts not only enhance our scientific understanding but can also inform broader applications in technology and policy.
Astrophysical Predictions
Astrophysical predictions involve the study of celestial bodies and their interactions. This field leverages both observational astronomy and theoretical physics to predict the behavior of planets, stars, galaxies, and cosmological events. The ability to forecast events such as supernovae, black hole formations, and gravitational waves has profound implications. These predictions enhance our knowledge of the universe, as well as our understanding of essential processes such as nucleosynthesis and cosmic expansion.
Astrophysicists utilize advanced models to analyze vast amounts of data collected from telescopes and space missions. For example, the predictions related to the behavior of dark matter and dark energy rely heavily on gravitational lensing effects that are only observable through meticulous calculations and simulations. More importantly, developments in technology have made it possible to predict the occurrence of exoplanets with increasing accuracy, thus opening doors to possible habitability studies in distant worlds.
"Astrophysical forecasts are not merely theoretical exercises; they could one day lead us to discover new habitable zones beyond our solar system."
Quantum Mechanics and Future Technologies
Quantum mechanics has emerged as a frontier that enables forecasting a myriad of future technologies. This branch of physics studies the behavior of matter and energy at the atomic and subatomic levels. Its principles underpin phenomena such as superposition and entanglement, which are essential for developing next-generation technologies.
The predictions derived from quantum mechanics extend to fields such as quantum computing, cryptography, and advanced materials science. For instance, scientists are now able to predict the performance of quantum computers based on the principles of quantum states. The ability to perform these predictions holds potential for creating vastly improved algorithms, which can outperform classical computers in specific tasks.
Moreover, the study of quantum mechanics facilitates innovations like quantum sensors that offer unprecedented precision in measurement. This technology not only transforms scientific research but also has practical applications in industries ranging from telecommunications to healthcare.
In summary, the future of forecasting in physics is bright and filled with promise. Through careful analysis of data and robust modeling techniques, physicists contribute to cross-disciplinary understandings that drive innovation and shape societal advancements.
Forecasting in Earth Sciences
Forecasting in Earth Sciences plays a crucial role in understanding and addressing some of the most pressing challenges faced by humanity. The applications of forecasting techniques within this domain significantly contribute to our ability to predict environmental changes and potential disasters. The insights gleaned from these forecasts guide policymakers, researchers, and the public in making informed decisions related to environmental management and disaster preparedness.
Climate Change Modeling
Climate change modeling is a primary focus within Earth Sciences forecasting. These models utilize various datasets to simulate Earth’s climate system response to different factors such as greenhouse gas emissions, land use change, and natural phenomena. One of the key benefits of climate change modeling is its capacity to project future climate scenarios under a range of assumptions.
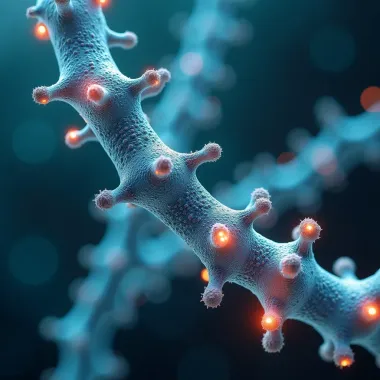
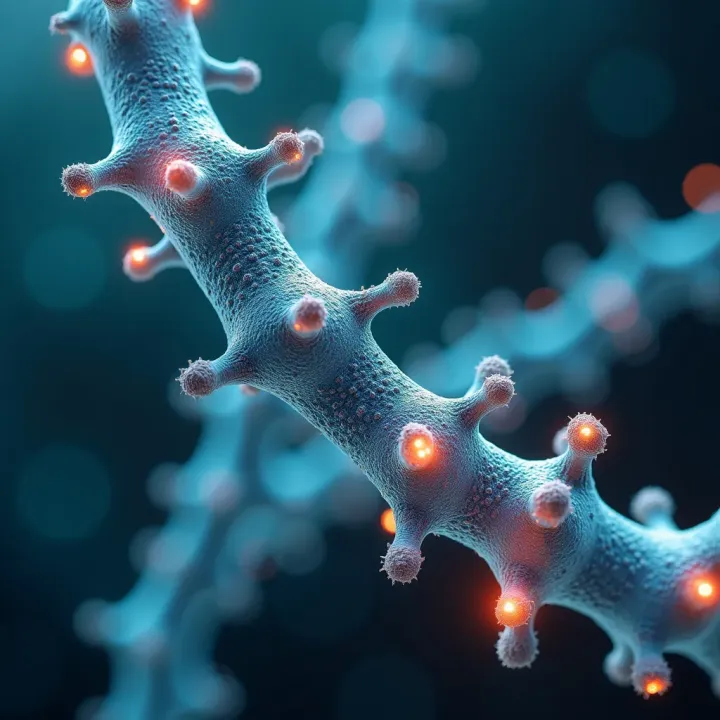
This forecasting approach allows researchers to assess possible outcomes and their implications for ecosystems, public health, and economies. For example, by developing models that predict temperature rises, rainfall patterns, and extreme weather events, scientists can enhance their understanding of the potential impacts of climate change on biodiversity, agriculture, and human settlements.
Moreover, policymakers can leverage this information to implement strategies aimed at mitigation and adaptation. As the frequency and intensity of climate-related events increase, the need for accurate and reliable climate change predictions becomes more crucial. The development of robust climate models remains an ongoing challenge, necessitating continuous advances in data collection and computational techniques.
Natural Disaster Predictions
Natural disaster predictions form another essential aspect of forecasting in Earth Sciences. The ability to predict events such as earthquakes, hurricanes, and floods can mitigate risks and enhance preparedness. For instance, sophisticated statistical models and machine learning techniques are used to analyze historical data and detect patterns that can indicate the likelihood of future disasters.
One of the major strengths of forecasting natural disasters lies in its potential to save lives and reduce economic losses. Early warning systems developed through accurate forecasting can inform communities of impending threats, allowing for timely evacuations and emergency preparations. However, predicting certain disasters, especially earthquakes, still presents significant scientific challenges due to the complex interactions within Earth’s crust.
Despite these challenges, advancements in geospatial technologies and real-time data analytics are improving our understanding of natural hazards. Ongoing research in this area can lead to better forecasting methods, ultimately enhancing resilience in vulnerable communities.
"Accurate predictions are not just numbers; they reflect the safety and readiness of entire communities."
Understanding forecasting in Earth Sciences makes clear its importance in shaping responses to environmental issues. By fostering interdisciplinary collaborations and integrating innovative technologies, we can strive for enhanced predictive capabilities that address both current and future challenges.
Challenges in Global Forecasting
Global forecasting serves as a crucial mechanism in understanding future outcomes across various domains. However, the task is not devoid of significant challenges. Addressing these challenges is essential for enhancing the accuracy and credibility of forecasts. The process of forecasting requires a rigorous analysis of data and methodologies. Given the complexity of global systems, a variety of factors can compromise the quality of predictions.
Among the many elements influencing forecasting are data limitations and the inherent uncertainties tied to predictions. These challenges are not merely technical hurdles; they resonate deeply within the disciplines of biology, chemistry, physics, and earth sciences. Understanding these difficulties is critical for researchers, policymakers, and anyone interested in reliable forecasting.
Data Limitations
Data limitations stand out as a significant hurdle in the field of forecasting. The information required for accurate predictions often lacks quality, accessibility, or even availability. This situation is particularly pronounced in developing regions, where data collection infrastructure might be inadequate. Consequently, forecasts based on insufficient or poor-quality data can lead to misguided conclusions.
Several factors contribute to data limitations:
- Incomplete Datasets: Many datasets only cover specific time frames or geographical areas, leaving significant gaps.
- Biases in Data Collection: Variations in data collection methods can introduce biases, skewing results.
- Technological Barriers: Not all regions or disciplines have access to modern data-collection technologies, impacting the overall quality of the forecast.
These issues highlight the necessity for improved data-gathering techniques and cooperative initiatives between disciplines. Collaboration can help create a more unified data approach, useful for accurate forecasting across multiple fields.
Uncertainty and Variability
Uncertainty and variability are intrinsic to the process of forecasting. They arise from various sources, including natural phenomena, human behavior, and limitations in models used for predictions. Acknowledging this uncertainty is important to avoid overreliance on predicted outcomes.
Key points regarding uncertainty include:
- Stochastic Nature of Systems: Many systems exhibit unpredictable behavior, making precise forecasting difficult.
- Model Limitations: Even state-of-the-art predictive models have inherent constraints. Simplifications may overlook critical interactions.
- Dynamic Variables: External factors, such as changing policies or environmental conditions, can introduce variability that is hard to anticipate.
"Understanding the limits of forecasting is as crucial as making predictions. It informs stakeholders on how to respond to potential outcomes."
Interdisciplinary Approaches to Forecasting
The field of global forecasting benefits immensely from interdisciplinary approaches. By integrating knowledge, methods, and practices from diverse disciplines, researchers can create more comprehensive and effective predictive models. The complexity of global challenges like climate change, health crises, and technological advancements necessitates similar integration to improve accuracy and relevance of projections. Utilizing an interdisciplinary lens enhances the ability to see interconnections between various phenomena that a single discipline may overlook.
Integrating Disciplines for Comprehensive Models
Integrating various disciplines allows for the development of models that reflect multidimensional aspects of real-world issues. For example, an effective model predicting the spread of infectious diseases combines biology, public health, and sociology. The biological aspects address environmental factors, while sociological insights provide understanding of human behavior in response to disease outbreaks. The collaboration ensures that the models reflect realities rather than theoretical assumptions.
Moreover, effective integration can lead to improved resource allocation and policy-making. When forecasts are supported by robust interdisciplinary data, stakeholders are more informed and can thereby make decisions that are timely and relevant.
Collaboration Across Fields
Collaboration across various fields of expertise is crucial in reinforcing the strength of interdisciplinary forecasting. This collaboration not only promotes sharing of data but also fosters innovation. When experts from diverse backgrounds work together, they often accomplish more than they could individually. This collaboration can take many forms:
- Joint Research Projects: Various institutions may engage in collaborative research that pools resources and knowledge from different disciplines.
- Workshops and Conferences: These events allow professionals to network, exchange ideas, and develop common frameworks which enhance understanding of complex problems.
- Academic Partnerships: Universities often establish interdisciplinary programs that focus on addressing global challenges through various academic lenses.
In summary, interdisciplinary approaches to forecasting present significant benefits. They yield models that are richer in detail and more relevant, thereby facilitating informed decision-making that can navigate the complexities of modern challenges. As the world continues to grapple with new and evolving issues, fostering collaboration across diverse fields will prove invaluable.
The Role of Technology in Forecasting
In contemporary forecasting, technology is the bedrock of accurate and effective predictions. The significance of technological integration across disciplines cannot be overstated. As we unfold the layers of forecasting methodologies, technology emerges as a pivotal player, enabling researchers to interpret complex datasets, analyze patterns, and derive predictive insights. Technology provides tools that enhance both the capability and the reliability of forecasting models, which, in turn, yield more precise projections.
Advancements in Computational Tools
The evolution of computational tools has revolutionized forecasting. Traditional methods often struggled with the vast amounts of data generated in various fields. Now, advanced algorithms and computational frameworks streamline the processing of this information. Technologies like cloud computing enhance accessibility and allow researchers to work with large datasets without significant local resource constraints.
Many forecasting models are now utilizing software that supports high-performance computing. This includes tools like Python with libraries such as Pandas and NumPy for data manipulation, and R for statistical analysis. These languages offer environments conducive to experimental forecasting, allowing for rapid iteration and testing of various hypotheses.
Advancements in computational tools have created an ecosystem where data scientists can engage with complex predictive models more effectively than ever before.
Additionally, visualization software aids in presenting the forecasts clearly. Tools like Tableau or Power BI transform raw data into meaningful visual representations. This clarity fosters better understanding among stakeholders and informs decision-making processes.
Big Data and Predictive Analytics
Big Data fundamentally alters how forecasts are generated. The volume of data available nowadays is unprecedented, coming from diverse sources such as social media, satellite imagery, and IoT devices. This wealth of information provides a treasure trove for predictive analytics. By employing machine learning algorithms, analysts can uncover correlations and anomalies that traditional methods might overlook.
The predictive analytics landscape thrives on techniques such as regression analysis, neural networks, and decision trees. These methods facilitate the extrapolation of data trends and patterns.
Some key considerations in leveraging Big Data include:
- Data Quality: Ensuring the data is relevant, accurate, and clean before analysis.
- Algorithm Selection: Choosing the right algorithm to best fit the data characteristics and the specific forecasting goals.
- Ethical Use of Data: Engaging with data responsibly to avoid pitfalls such as bias in predictive models.
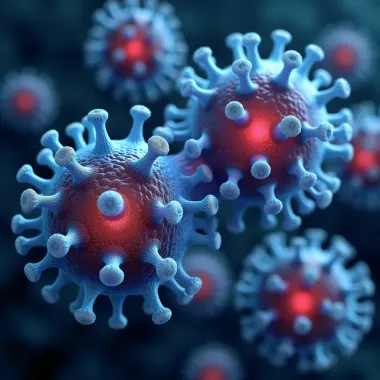
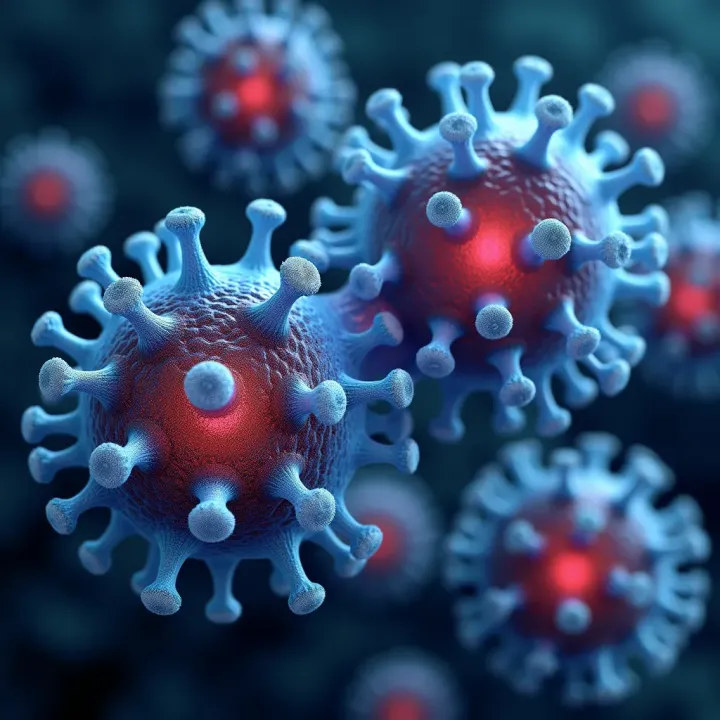
As organizations increasingly rely on big data, the implications for accuracy and strategy become more substantial. Enhanced predictive capabilities lead to better-informed decisions on a corporate and governmental level, impacting policy-making and resource management.
Future Directions in Global Forecasting
The realm of global forecasting is at a pivotal juncture, with rapid advancements shaping its future trajectory. Understanding these future directions is crucial not just for researchers, but also for policy makers and society at large. The pathways of progress in forecasting methodologies offer insights into how societies will tackle complex challenges, from climate change to health crises. Predictions are more than just educated guesses; they are foundational to strategic decision-making and planning.
As we explore the future of forecasting, we must consider several key elements. There is a need to integrate diverse data sources more effectively. This integration can enhance the reliability of predictions and can provide a more comprehensive perspective on the issues at hand. Moreover, as computational power continues to grow, the potential for more sophisticated models becomes increasingly tangible.
The implications of emerging trends in forecasting are profound, revealing the critical relationship between data and decision-making.
Emerging Trends
Several emerging trends are shaping the landscape of global forecasting. One significant trend is the rise of artificial intelligence. AI enhances the ability to process vast amounts of data, providing more accurate predictions across various fields, such as climate science and public health. Machine learning algorithms are becoming increasingly popular, helping to unravel the complexities within diverse datasets.
Another trend is the increasing significance of interdisciplinary approaches. Collaborative efforts among different fields can lead to more holistic forecasting models. For instance, combining insights from biology and environmental science can yield better understanding of ecosystem changes and their impacts on biodiversity. With the proliferation of open data initiatives, there is more opportunity for researchers to collaborate, share data, and refine their models more efficiently.
Finally, global connectivity plays a pivotal role in forecasting. Real-time data access allows for agility in responses to emerging situations, making it essential for effective risk management.
Implications for Policy and Society
The implications of advancements in forecasting extend deeply into policy formation and societal change. Policymakers rely heavily on accurate forecasting to craft responses to pressing issues, such as healthcare inequalities and climate change risks. Effective projections enable authorities to allocate resources more judiciously and develop proactive strategies.
Furthermore, understanding projections supports informed public discourse. When citizens comprehend the basis of forecasts, they are more likely to engage in discussions about relevant policies and adapt their behaviors accordingly. Strengthening public trust in forecasts is essential to foster cooperative efforts in sectors ranging from environmental sustainability to disaster preparedness.
Case Studies of Successful Forecasting
Case studies provide practical examples of forecasting in action. They illustrate the real-world application of various methodologies, highlighting what works and what does not. This section will examine successful instances in biological and chemical forecasting, demonstrating the benefits derived from accurate predictions. Through detailed analysis, we can discern key factors contributing to the effectiveness of these case studies.
Biological Forecasting Successes
In the field of biology, successful forecasting has made significant impacts on public health and environmental conservation. One notable example is the forecasting of disease outbreaks. Models developed using historical data have allowed for accurate predictions of influenza's seasonal patterns. Researchers have utilized data from previous years to create robust models. These models can forecast potential spikes in infection rates, assisting public health officials in resource allocation and awareness campaigns.
Another case is the prediction of species population dynamics. For instance, conservationists often use ecological models to predict the effects of climate change on endangered species. By analyzing data on species habitat and reproductive patterns, these models provide valuable insights. Conservation strategies can be developed in advance, leading to informed decisions that enhance species survival rates and ecosystem health.
- Key Benefits:
- Improved decision-making in health management.
- Advanced preparation for environmental changes.
- Informed conservation strategies that leverage predictive insights.
Successful biological forecasting thus not only addresses immediate needs but also enhances long-term strategic planning across various ecological scenarios.
Chemical Innovations and Projections
In chemistry, case studies of forecasting illustrate the potential for innovation and improvement in material sciences. One significant example is the development of novel catalysts. Through systematic studies of reaction kinetics and thermodynamics, researchers have been able to predict the behavior of chemical reactions. Companies like BASF have pioneered advances by employing such forecasting techniques to develop catalysts that optimize processes. This innovation has contributed to enhanced efficiency in chemical production and minimized waste.
Moreover, predictive models aid in the development of new materials. By utilizing machine learning algorithms, researchers can predict materials' properties without extensive lab testing. The result is a faster, more efficient approach to material science. For instance, technologies like 3D printing have benefited from predictive methods that identify optimal material compositions for specific applications.
- Considerations:
- The accuracy of forecasts affects innovation pathways.
- Collaboration between fields can yield hybrid materials and processes.
Forecasting in chemistry showcases the ability to merge analysis with innovation, leading to tangible advancements in various industries. The implications of these case studies are profound, shedding light on how structured predictive methodologies can drive progress in real-world applications.
Ethical Considerations in Forecasting
Ethical considerations in forecasting are vital. They shape the ways predictions influence decisions and actions across various sectors. The implications of forecasts can extend beyond statistics; they can alter lives, ecosystems, and economies. Understanding these ethical frameworks helps ensure responsible use of predictive modeling techniques.
Responsibility in Predictive Modeling
Predictive modeling carries a significant weight of responsibility. It is important that those who conduct forecasts understand the potential consequences of their work. This requires integrity and transparency in methodologies. When models produce results that inform public policy, they must not only be accurate but also fair. The people involved in predictive analysis must ensure that their methods do not contribute to misinformation.
Additionally, being responsible involves taking into account bias in the data used. Data can reflect historical inequalities. If predictive models utilize biased data sets, the results can perpetuate harmful stereotypes or inequalities. Therefore, it is critical to assess the data critically and adjust models to mitigate these biases. This demands ongoing reflection on what the output implies for different groups within society. Predictive modeling must pursue ethical rigor to maintain trust among stakeholders.
Impact of Forecasts on Public Perception
Forecasting also significantly influences public perception. When a prediction is released, it forms public opinion almost instantaneously. This can lead to fear or complacency. For example, a report predicting severe climate change impacts can spur action or, conversely, create panic if not communicated properly. What this shows is that the framing of forecasts is essential. The language and context used in public-facing forecasts can shape reactions.
Furthermore, misinformation or exaggerated forecasts can lead to erosion of trust in scientific institutions. It may create a divide between scientists and the public, where the latter feels disconnected from the methodologies used in forecasting. Researchers must communicate their findings in informed and accessible ways to prevent misunderstandings.
In summary, ethical forecasting demands transparency and responsibility. Public trust hinges on clear, accurate communication that considers the potential societal impacts of predictive models. Understanding these dynamics plays a crucial role in the future of forecasting across disciplines.
Ensuring the ethical application of forecasting can lead to better decision-making, ultimately enriching the human experience across various domains.
Epilogue
The conclusion serves as the epitome of understanding gained through the exploration of global forecasting. Throughout this article, the essential role of interdisciplinary collaboration in enhancing predictive accuracy has been emphasized. Each discipline, from biology to chemistry and physics, contributes unique insights, enriching the overall framework of forecasts. This is crucial for addressing complex issues society faces today, such as climate change, health crises, and technological advancements.
Summary of Key Insights
In summary, several key insights emerge from our discussion:
- Importance of Methodologies: Different methods, whether qualitative or quantitative, shape the accuracy and reliability of forecasts.
- Interdisciplinary Approaches: Collaboration across various scientific domains enhances the robustness of global predictions.
- Ethical Considerations: The moral implications of forecasting cannot be overlooked, as forecasts influence public perception and policy decisions.
These insights reiterate the value of integrating perspectives from diverse fields to comprehend the implications of projected events.
The Future of Global Forecasting
Looking ahead, the future of global forecasting appears promising, yet it is fraught with challenges. Emerging technologies like artificial intelligence and big data analytics are expected to transform predictive capabilities. Researchers are likely to develop more refined models that can account for the variability and uncertainty present in global data.
- Advancements in Data Processing: Increased computational power will enable the analysis of vast datasets, improving the speed and accuracy of forecasts.
- Focus on Sustainability: As global challenges become more urgent, forecasting will increasingly emphasize sustainability and resilience in its projections.
- Public Engagement: Improved communication of forecasts to the public can enhance understanding and preparedness in various sectors.
The trajectory of global forecasting will be shaped by these trends, making it an essential field for future inquiries and applications. Understanding these dynamics will allow stakeholders to make more informed decisions, ultimately leading to better outcomes for society.